Understanding SPSS: A Comprehensive Analytical Guide
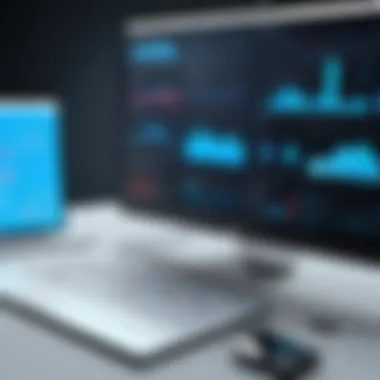
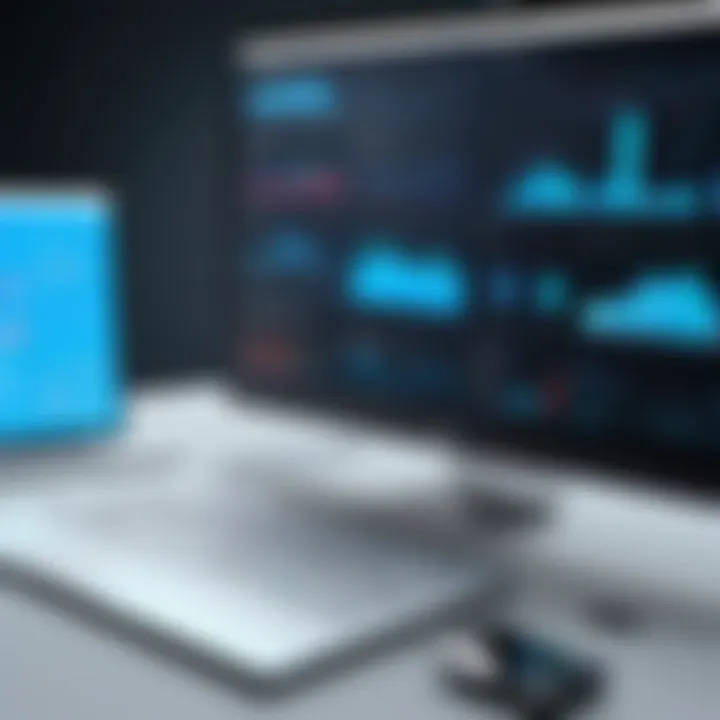
Intro
SPSS, or Statistical Package for the Social Sciences, has been a mainstay in data analytics since its inception in the late 1960s. Its strong reputation stems from its accessible user interface and robust statistical capabilities. SPSS serves as a valuable resource across various sectors including academia, healthcare, and market research. With its continuous development, it addresses ever-evolving analytical demands.
In this guide, we will thoroughly explore the fundamental aspects of SPSS. The focus will be on its functionalities, the benefits of using it, and how it compares with other analytical tools. From discussing pricing models to user experiences, each detail will serve to illuminate the significant roles of SPSS in data analysis today.
Preamble to SPSS Analytical Software
SPSS has become a crucial tool in the realm of data analysis. Its ability to handle large datasets and perform complex statistical procedures positions it as a key asset for researchers and professionals. Understanding SPSS is not only about knowing how to use the software but recognizing its impact in various sectors. Efficient data management leads to informed decisions, which is essential in today’s data-driven world.
Definition of SPSS
SPSS stands for Statistical Package for the Social Sciences. It is a software product developed by IBM that specializes in statistical analysis. Originally created for social science research, it has broadened its scope to serve many fields, including market research, healthcare, and education. The software simplifies the process of data input, manipulation, and statistical modeling, making it accessible to users with varying levels of expertise.
Historical Background
The journey of SPSS began in 1968 when Norman H. Nie, C. Hadlai Hull, and William G. Harder developed the software at Stanford University. Initially, it focused on aiding social scientists in analyzing survey data. Over the years, SPSS evolved significantly. In 2009, IBM acquired it, accelerating its development and integration with other software tools. This acquisition expanded its functionalities, positioning it as a market leader in analytical software.
Evolution of SPSS Software
SPSS has undergone several transformations since its inception. Initially, it operated in a command-line interface, which was less user-friendly. As technology evolved, the user interface improved, adding graphical tools and a user-friendly layout. The introduction of SPSS Statistics enabled users to access advanced statistical techniques without extensive programming knowledge. Furthermore, regular updates have incorporated features like advanced data visualization, machine learning capabilities, and enhanced data management tools. Each iteration reflects a response to user needs and technological advancements, solidifying SPSS's status in data analysis.
Core Functionalities of SPSS
The core functionalities of SPSS are central to its effectiveness as a data analysis tool. Understanding these features is crucial for users who aim to leverage data insightfully. SPSS offers robust capabilities that enable users to manipulate data, perform statistical analyses, and create visual representations of findings. This section will detail the three key areas of SPSS's core functionalities: Data Management, Statistical Analysis Tools, and Data Visualization Capabilities.
Data Management
Data management in SPSS is a critical component of the software’s functionalities. It facilitates the efficient organization, manipulation, and preparation of data for analysis. Users can import data from various sources such as spreadsheets and databases, enabling seamless integration of information.
Additionally, SPSS allows for data cleaning and preprocessing. This includes identifying and addressing missing values, filtering out unnecessary entries, and restructuring datasets. This not only ensures the quality of data but also enhances the reliability of analysis conducted afterward. It is essential for researchers and analysts to understand how to manage data effectively, creating a solid foundation for subsequent statistical evaluations.
Statistical Analysis Tools
Statistical analysis tools in SPSS are extensive and versatile. With options ranging from basic statistical tests to complex modeling techniques, SPSS caters to a wide range of analytical needs. Users can perform descriptive statistics, inferential tests, regression analysis, and multivariate analysis. The software's user-friendly interface simplifies the execution of these statistical tests.
Moreover, SPSS includes features for hypothesis testing and significance testing, which are essential in many research areas. Users benefit from the ability to conduct t-tests, ANOVA, chi-square tests, and more. Such tools are indispensable in deriving meaningful conclusions from data, allowing researchers to validate their hypotheses or uncover new insights.
"Statistical analysis is the backbone of data interpretation, and SPSS shines in this arena with its comprehensive tools."
Data Visualization Capabilities
Data visualization capabilities in SPSS significantly enhance the interpretability of data. Through various graphical representations such as histograms, bar charts, scatter plots, and box plots, users can visually analyze trends and patterns in data. These visualizations help in understanding complex information more easily than raw data would allow.
Furthermore, SPSS supports customizable charts and visual outputs. As a result, users can tailor their visual communications to effectively convey findings to different audiences. The ability to produce high-quality graphics is an essential asset in both academica and professional settings, where presenting data in an accessible way is key.
In summary, the core functionalities of SPSS encompass a broad spectrum of essential capabilities. From managing and cleaning data to executing diverse statistical analyses and visualizing results, these features collectively empower users to derive implications from their data. Understanding these functionalities is crucial to harness SPSS's full potential in various analytical endeavors.
Applications of SPSS in Various Domains
SPSS software plays a significant role in numerous sectors due to its analytical capabilities. The diverse applications of SPSS demonstrate its flexibility and relevance in data analysis across academic, commercial, and governmental fields. Each domain utilizes SPSS differently, tailoring its features to meet specific needs. Understanding these applications can help users grasp the broader impact of SPSS in various contexts.
Academic Research
In academic research, SPSS serves as a fundamental tool for data analysis. Researchers rely on its extensive collection of statistical functions to derive insights from data sets. They can perform various analyses, including descriptive statistics, inferential statistics, or hypothesis testing. This software allows researchers to validate their findings methodically, enhancing the credibility of their work.
Additionally, SPSS encourages collaboration among researchers. Since many academics are familiar with its interface, they can easily share data and findings. Moreover, its compatibility with other software systems enables efficient data integration, which is crucial for larger research projects.
Market Research
Market research professionals utilize SPSS to collect and analyze consumer data. Understanding market trends is vital for businesses, and SPSS provides the tools necessary for comprehensive analysis. It assists researchers in designing surveys, analyzing responses, and producing visual representations of data. This systematic approach helps organizations make informed decisions about products, services, and target audiences.
Moreover, SPSS enables segmentation analysis, allowing marketers to identify and target specific customer groups. This capability enhances marketing strategies, ensuring campaigns are efficient and focused on the right demographics. By analyzing purchase patterns and customer behavior, companies can adapt strategies according to consumer needs.
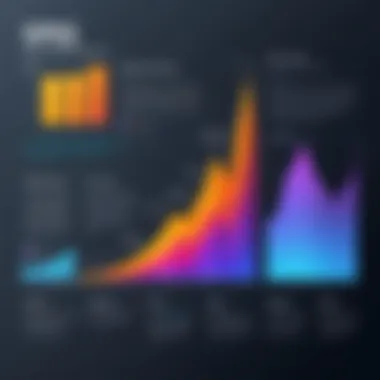
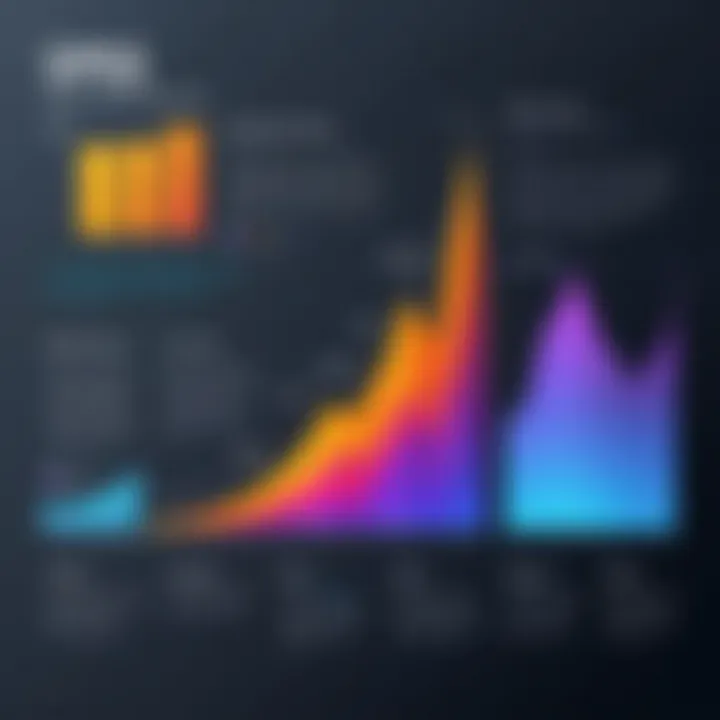
Healthcare Analytics
In the healthcare sector, SPSS is instrumental in analyzing patient data and treatment outcomes. The ability to track and evaluate patient conditions over time helps healthcare providers improve treatment. SPSS can analyze large data sets, revealing trends in health issues and treatment efficacy. This information is vital for improving patient care and validating medical procedures.
Healthcare analysts can also perform predictive modeling with SPSS, forecasting patient admissions or potential health outbreaks. This predictive capacity aids in resource allocation, ensuring facilities are prepared for varying demands. Overall, SPSS enhances the ability of healthcare organizations to use data effectively for better patient outcomes.
Government and Public Policy
Governments and public policy makers depend on SPSS for data-driven decisions. They analyze demographic data, economic statistics, and social trends with the software. This analysis informs policy decisions and helps craft effective programs that address public needs.
SPSS assists in evaluating the impact of policies and programs over time. By analyzing data collected from various sources, analysts can assess effectiveness and adjust strategies accordingly. This evidence-based approach enhances transparency and accountability in government actions.
Advanced Techniques in SPSS
The advanced techniques in SPSS are essential for users aiming to conduct in-depth data analysis. These methods dive beyond basic statistical functions, allowing for more complex and multifaceted exploration of data sets. Understanding regression analysis, factor analysis, and cluster analysis provides valuable insights into relationships within data, patterns in user behavior, and segments in customer groups. Each of these techniques serves distinct purposes and is crucial for making informed decisions based on data.
Regression Analysis
Regression analysis is a powerful statistical tool used to investigate relationships between variables. It helps predict the value of a dependent variable based on one or more independent variables. This technique is essential for those engaged in academic research or industry analytics as it enables users to quantify relationships mathematically. The results can guide strategic choices, including marketing efforts or resource allocation.
An important consideration in using regression is ensuring that the underlying assumptions are met. For instance, linear regression requires that the relationship between variables is linear and that the residuals (differences between observed and predicted values) are normally distributed. Proper diagnostics are essential to verify these conditions.
Benefits of regression analysis include clarity in predicting outcomes and understanding how each predictor influences the response variable.
Factor Analysis
Factor analysis is another sophisticated technique found in SPSS. It is primarily used to identify underlying relationships between variables. By reducing the number of variables into a smaller set of factors, researchers can simplify their analyses. This method is common in psychology and market research, allowing analysts to identify latent variables that explain the variance in various observable data points.
Factor analysis produces factors that encapsulate common themes among selected variables. This aids in interpreting complex data while minimizing potential noise from less relevant data. Users must exercise caution, as improper use of factor analysis can lead to misleading interpretations. For this reason, a solid understanding of the technique's foundational concepts is necessary.
Cluster Analysis
Cluster analysis is a classification method that groups a set of objects in such a way that objects in the same group (or cluster) are more similar to each other than to those in other groups. This technique is highly relevant in market segmentation, social science research, and biological classification.
By applying cluster analysis in SPSS, organizations can find natural groupings in data, enhancing their targeting efforts. For instance, companies can identify segments within their customer base, allowing for tailored marketing strategies. However, the interpretation of clusters can sometimes be subjective. Thus, it is paramount to validate the clusters against known benchmarks or external criteria to ascertain their effectiveness.
Understanding these advanced techniques in SPSS equips users with tools to unlock deeper insights from their data, ultimately guiding decision-making processes and strategy development.
"Advanced techniques in SPSS broaden analytical capabilities, paving the way for nuanced understanding in diverse fields."
User Experience and Interface
In today’s digital landscape, a seamless user experience is paramount, especially in analytical software like SPSS. Users come from various backgrounds, and their proficiency levels can vary greatly. Hence, the interface design should be intuitive yet powerful, catering to the needs of both novice and advanced users. Understanding the user experience in SPSS allows users to leverage the software's full potential efficiently. A well-designed interface simplifies complex functionalities, thus enhancing productivity and minimizing frustration.
Navigating the SPSS Interface
The SPSS interface is designed with multiple menus, toolbars, and a data viewer that enables effective navigation. Users can easily access data management, statistical tests, and graphical outputs from a centralized location. The main components of the interface include:
- Data View: This is where users can input and edit their dataset. Each column represents a variable, while each row corresponds to a case or observation.
- Variable View: This view allows users to define properties of variables, such as names, types, and measurement levels.
- Output Viewer: After running analyses, results display in the output viewer. This organized space allows for easy review of results, tables, and charts.
Navigating these components is straightforward. Tabs located in the main window grant quick access to all essential functionalities. Users should familiarize themselves with these elements for a smooth experience within SPSS. Moreover, keyboard shortcuts can be handy for navigating around quickly, saving valuable time.
Tips for Efficient Use
Efficiency in SPSS can significantly enhance the analytical process. Here are some practical tips:
- Stay Organized: Structuring datasets before importing them into SPSS can minimize errors and streamline processes.
- Utilize Syntax: Although SPSS provides a graphical user interface, developing familiarity with its syntax can lead to greater control and automation.
- Leverage Help Resources: SPSS comes with extensive documentation. Users should explore these resources to troubleshoot issues or learn about advanced features. Online forums, such as Reddit, offer community support and discussions that can prove useful.
- Practice Regularly: Familiarity with the software grows through consistent use. Regular practice helps users remember where to find necessary tools and features.
"Mastering the user interface of SPSS is essential to perform analyses effectively, facilitating deeper insights through data interpretation."
By implementing these strategies, users will find their experience with SPSS more productive. This sets a solid foundation for maximizing the software’s capabilities, especially in complex analytical tasks.
Integration with Other Software
Integration with other software is a critical component of SPSS, enhancing its overall functionality and utility in data analysis. This integration allows users to streamline their workflow, improve efficiency, and access a broader range of tools and data sources. As a data analysis platform, SPSS is often not used in isolation; instead, it works in conjunction with various other applications, making it vital for users to understand how to effectively connect and utilize these systems.
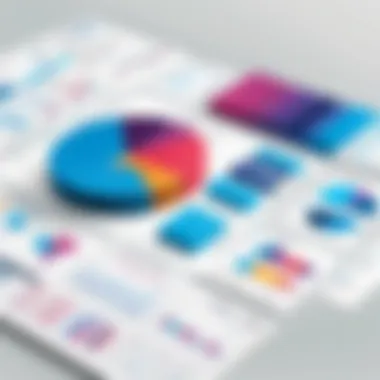
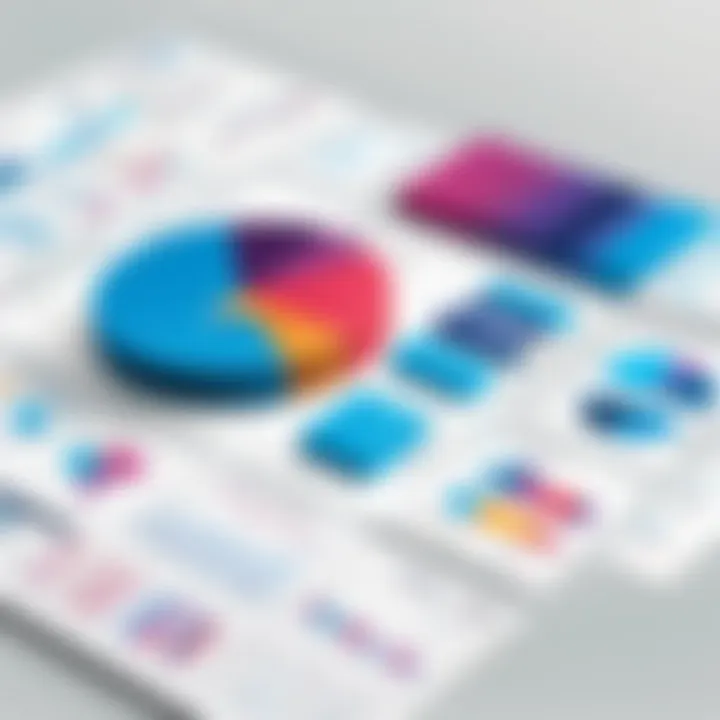
Connecting to Databases
SPSS supports connections to various databases, which enables users to import data directly for analysis. Common databases include Microsoft SQL Server, Oracle Database, and IBM Db2. Connecting to databases simplifies the import process, ensuring that analysts can work with current and comprehensive data without the need for manual data entry or export.
When connecting to a database, users must first ensure that they have the necessary access permissions. Once access is granted, they can utilize the Database Wizard in SPSS to establish a connection. This involves selecting the appropriate database type, entering the required credentials, and choosing the data tables needed for analysis. The process is relatively straightforward and can be completed in just a few steps.
Additionally, once connected, users can run SQL queries directly within SPSS to filter data as needed. This ability to manipulate and access data directly within the analysis software significantly enhances the analytical capabilities available to writers and researchers.
Importing Data from Other Sources
In addition to direct database connections, SPSS allows for the importation of data from various other sources. This includes data from spreadsheets, text files, and even specific statistical packages. Common formats for import include CSV, Excel, and plain text files. The ability to import diverse data formats is crucial for analysts who need to combine different datasets or integrate findings from other analytical tools.
To import data into SPSS, users can start by navigating to the "File" menu and selecting "Import Data". This process guides users through selecting the correct file and configuring necessary import settings. One essential setting is ensuring the imported columns align correctly with the intended variables in SPSS. This consideration helps maintain the integrity and structure of the data throughout the analysis process.
In summary, the integration capabilities of SPSS with other software allow users to maximize their analytical potential. Connecting to databases and importing data from various sources streamlines data management and empowers users to leverage a wide array of inputs for informed decision-making. This adaptability is critical in today's data-driven environment, where merging insights from multiple platforms can lead to significant breakthroughs.
Security and Data Privacy in SPSS
In today’s data-driven world, the significance of security and data privacy in analytical software cannot be understated. SPSS, or Statistical Package for the Social Sciences, often handles sensitive information across various domains such as healthcare and market research. This means robust security measures are essential not only for compliance but also for maintaining user trust. Data breaches can lead to severe reputational damage and legal consequences, making it imperative for users to be aware of the security features that SPSS offers.
Data Encryption Features
Data encryption is a critical element of protecting sensitive information. SPSS incorporates advanced encryption techniques to ensure that data is stored and transmitted securely. Encryption transforms readable data into an encoded format that can only be accessed by those with the proper decryption keys. This is particularly relevant for organizations handling personal or sensitive data, as encrypted data remains protected even if unauthorized access occurs.
In SPSS, users can implement encryption at multiple levels. For instance, file encryption ensures that datasets saved on computers or remote storage are not accessible without the correct authorization. Additionally, secure communication protocols can encrypt data transmitted through the internet. Understanding and utilizing these features effectively builds a robust defensive layer against potential data breaches.
User Access Controls
User access controls are equally important in maintaining the integrity and confidentiality of data within SPSS. These controls determine who can access the software, what data they can see, and what actions they are allowed to perform. SPSS allows the configuration of different user roles, each with specific permissions. This means organizations can restrict sensitive data to authorized personnel only, thereby minimizing the risk of data leaks.
The implementation of user access controls involves setting up unique login credentials for each user. This ensures accountability, as actions within the software are traceable to individual accounts. Moreover, organizations can monitor logins and user activity to detect any suspicious behavior. Regular audits of access rights can also help ensure that outdated permissions are updated or removed as needed.
In summary, the security and data privacy features offered by SPSS, such as data encryption and user access controls, are indispensable for ensuring the safe handling of data. Organizations must leverage these capabilities to fortify their data protection strategies and enhance their overall reliability.
"Data privacy is not an option; it is a necessity."
Each feature plays a role in creating a secure environment for data analysis, fostering a trustworthy relationship with users and stakeholders.
Cost and Licensing Options
Understanding the costs associated with SPSS is crucial for potential users, especially for businesses and academic institutions. The cost of the software can impact decision-making processes regarding its adoption. Analyzing the various licensing models available helps users to assess their options based on budget constraints and usage requirements. Different pricing structures offer flexibility and cater to diverse needs, making it essential to consider these factors thoroughly before committing to a purchase.
Pricing Structure
The pricing structure for SPSS typically depends on the type of license purchased. Users can choose between a subscription model or a perpetual license. A subscription model allows users to access the latest version of SPSS for a specified period, usually billed monthly or annually. This option tends to be more affordable in the short term and is suitable for individuals or organizations needing temporary access to the software.
On the other hand, a perpetual license requires a one-time payment for indefinite use of a specific version, but it may involve additional costs for future upgrades.
Besides these primary options, various add-on packages are often available to enhance functionality, such as predictive analytics or data visualization tools. It is also prudent to keep in mind any potential ongoing maintenance fees, which may arise depending on the license acquired.
Student and Academic Licenses
SPSS recognizes the need for affordable access to its powerful analytical capabilities for students and educational institutions. To facilitate this, student and academic licenses are offered at significantly reduced prices compared to regular licenses. These licenses enable learners to utilize SPSS for their coursework, projects, and research, providing them with exposure to essential tools in data analysis.
Eligibility for student licenses often requires verification through educational institutions, ensuring only students can benefit from these discounts. Furthermore, academic institutions may inquire about site licenses that provide access to all students or faculty members, making it a cost-effective solution for comprehensive educational programs.
"Choosing the right licensing model can save organizations significant resources in the long run."
Comparison with Other Analytical Software
In the realm of data analysis, understanding how SPSS stacks up against its competitors is crucial for informed decision-making. Analytical software varies in features, user experience, and suitability for different tasks and industries. Therefore, a thorough comparison can assist users in selecting the appropriate tools for their unique needs. SPSS, being a leading software, presents distinct advantages and some limitations that can significantly influence its usability.
Advantages of SPSS
SPSS offers several compelling advantages that contribute to its popularity among researchers and analysts:
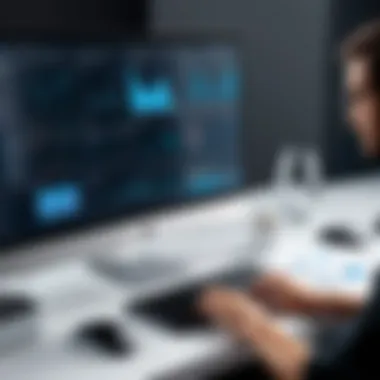
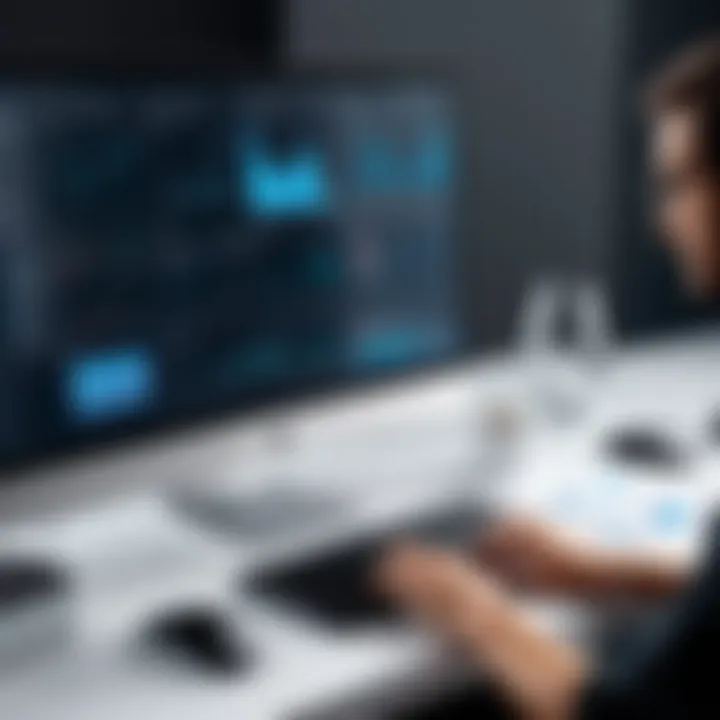
- User-Friendly Interface: SPSS is known for its intuitive design, which simplifies the process of data entry and analysis. Users with limited statistical background can navigate its features more easily compared to more complex software.
- Comprehensive Statistical Tools: The software includes a broad array of statistical functions, from basic descriptive statistics to advanced modeling techniques. This ensures that users can carry out various analyses without needing multiple packages.
- Data Handling Capabilities: SPSS excels in managing large datasets. It efficiently handles missing data and offers numerous data transformation options, making it easier for users to prepare their data for analysis.
- Extensive Documentation and Support: With a long history, there is a wealth of resources available for SPSS users. Online forums, official documentation, and tutorials provide ample opportunities for learning and problem-solving.
Despite these advantages, there are certain drawbacks to consider as well.
Disadvantages of SPSS
While SPSS has many benefits, it also has some disadvantages that bubble to the surface:
- Cost: One notable drawback is the expense. SPSS can be cost-prohibitive for some users, especially students and small businesses. The licensing fees may restrict its accessibility compared to open-source alternatives.
- Limited Customization: Users may find SPSS somewhat rigid regarding tailoring certain functions. Unlike programming-based tools such as R or Python, SPSS does not offer the same level of flexibility in customizing analyses.
- Steep Learning Curve for Advanced Features: While basic functionalities are easy to use, mastering the more complex statistical methods may be challenging. Users often need to invest significant time to become proficient in the advanced analytical tools within SPSS.
Popular Alternatives to SPSS
When considering alternatives to SPSS, several options stand out:
- R: A powerful open-source programming language that offers extensive statistical capabilities and customization options. It is a favorite among statisticians and data scientists for its versatility.
- Stata: Another well-known statistical software package, Stata specializes in data management and advanced analysis. It has a firm following in academia and economics.
- Python with Libraries: Python, combined with libraries like Pandas and SciPy, provides an open-source option with a wide range of applications in data analysis and machine learning.
- Microsoft Excel: For simpler data analysis tasks, many users turn to Excel. While not as advanced as SPSS, its accessibility makes it a common starting point for many.
Understanding these comparisons allows analysts and organizations to make strategic decisions in choosing the right analytical software for their requirements.
Future of SPSS and Data Analysis
The future of SPSS and data analysis lies at the intersection of evolving technologies and increasing data complexity. As businesses and researchers seek insights from larger datasets, the importance of robust analytical tools like SPSS becomes ever more pronounced. This section will delve into emerging trends in data analytics and the role of machine learning within SPSS, illustrating how these elements shape the future landscape of data analysis.
Emerging Trends in Data Analytics
Data analytics is constantly changing. Current trends prioritize automation, cloud computing, and real-time data processing. As organizations generate massive amounts of data, traditional methods are not sufficient. Here's how emerging trends influence SPSS:
- Cloud Integration: Many businesses are shifting to cloud-based analytics. SPSS is increasingly being integrated with cloud services, allowing for easier access, collaboration, and scalability. This shift enhances computational power without the necessity for extensive local infrastructure.
- Data Democratization: There is a growing movement towards making data accessible to non-technical users. SPSS has begun incorporating user-friendly interfaces and self-service analytics features. This trend empowers a wider audience to engage with data analysis.
- Real-Time Analytics: Rapid decision-making is crucial. The ability to analyze data in real-time is becoming essential. SPSS is adapting by adding capabilities that allow users to conduct analysis on streaming data, improving responsiveness in business environments.
- Big Data Capabilities: The tools available within SPSS are evolving to handle large volumes of unstructured and structured data. Data from social media, IoT devices, and other sources can now be analyzed efficiently, expanding the analytical scope.
"The future of data analytics is not only about the volume of data, but about how quickly and efficiently we can turn this data into actionable insights."
Role of Machine Learning in SPSS
Machine learning is transforming how data is analyzed and understood. In the context of SPSS, machine learning provides several advantages that enhance traditional statistical analyses:
- Predictive Analytics: With machine learning algorithms, SPSS can make more accurate predictions based on historical data. This capability is beneficial for sectors such as finance and healthcare, where anticipating trends and behaviors is vital.
- Automated Insights: Machine learning automation in SPSS reduces the manual effort required in data analysis. By automatically identifying patterns and correlations, this technology allows analysts to focus on strategic decision-making activities.
- Model Validation: Machine learning techniques can validate models more rigorously than traditional methods. This leads to enhanced reliability and confidence in the results generated from SPSS.
- Natural Language Processing: With the rise of unstructured data, SPSS is exploring natural language processing. This integration aids in the interpretation of text data, which can be particularly valuable in qualitative research and sentiment analysis.
As SPSS embraces these trends and advancements, it ensures its relevance in a field that demands agility and responsiveness to ever-changing data landscapes. Understanding these aspects can help users leverage SPSS effectively for future data-driven challenges.
Training and Resources for SPSS Users
Training and resources for SPSS users play a critical role in one’s ability to leverage this analytical software effectively. The depth and breadth of SPSS functionalities require a solid understanding for proficient application in various settings. Without proper training, users may not fully exploit SPSS capabilities, which can lead to uninformed decisions or inadequate analyses. Thus, investing time in the right resources can significantly impact the overall quality of data interpretations.
Several considerations underlie the importance of these training mechanisms. Firstly, online courses often provide structured learning, guiding users from basic concepts to more advanced applications. In a field so reliant on precision and accuracy, such clarity is invaluable. Secondly, the availability of certifications validates one’s knowledge, enhancing professional credibility. Lastly, continuous learning through user communities and forums not only keeps users updated on the latest trends but also provides a support network for troubleshooting and sharing best practices.
Online Courses and Certifications
The proliferation of online courses has made it easier than ever for users to start their SPSS journey. Many reputable platforms offer courses specifically designed to cater to different levels of expertise. Users can begin with introductory courses that familiarize them with the SPSS interface, guiding them through fundamental data management techniques and basic statistical analyses. Following these foundations, intermediate and advanced courses introduce users to more complex methodologies such as regression and factor analysis.
Some notable platforms providing SPSS courses include Coursera, Udemy, and LinkedIn Learning. Each of these platforms frequently updates their content to ensure that learners receive the most current information and tools available. Moreover, many of these courses come with certifications upon completion, which can be advantageous for those seeking to enhance their resumes. This adds a layer of motivation, as users can concretely measure their progress and showcase their skills to potential employers.
"Certification in SPSS can serve not only as an acknowledgment of skill but also as a competitive edge in the job market."
User Communities and Forums
Engagement in user communities and forums is essential for SPSS users aiming to enhance their practical understanding of the software. Online forums provide spaces for users to ask questions and share solutions. Platforms like Reddit host various groups focused on statistical analysis, where users can seek advice from peers and experts alike. Discussions may range from troubleshooting specific problems to sharing innovative analytical techniques or strategies.
These communities also offer users an avenue to stay updated about software updates, new features, and best practices. Participation in forums encourages continuous learning. Furthermore, connecting with peers can lead to collaborations that may provide fresh insights or alternative approaches to analysis tasks. Overall, being part of an active community can enrich a user’s experience and effectiveness with SPSS.
Ending
The conclusion serves as an essential component of this article. It offers an opportunity to reflect on the key points discussed and to emphasize the significance of SPSS in today’s analytical landscape. By summarizing major themes and insights, the conclusion consolidates the reader’s understanding of how SPSS functions and its value in various domains.
Summarizing Key Takeaways
In this article, we have explored various aspects of SPSS analytical software, underlining its features, benefits, and applications across multiple industries. Here are the key points to remember:
- Historical Context: Understanding the background of SPSS helps appreciate its evolution and ongoing relevance in modern analytics.
- Core Functionalities: We discussed vital features such as data management, statistical analysis tools, and visualization capabilities that make SPSS a preferred choice for many users.
- Application Domains: SPSS holds significant importance in academic research, market analysis, healthcare, and government sectors, showcasing its versatility.
- Advanced Techniques: Techniques like regression analysis and factor analysis enable users to derive deeper insights from data sets.
- User Experience: The interface is designed for ease of navigation, which is crucial for users requiring efficient data handling and analysis.
- Integration: SPSS’s ability to connect with other software further enhances its utility and user experience.
- Cost Considerations: Prospective users should evaluate pricing plans and available licenses, ensuring they align with their needs.
- Future Trends: The discussion on emerging trends and machine learning indicates SPSS's adaptability and potential in a rapidly evolving analytical environment.
"The value of SPSS lies not just in its features, but in how it empowers users to unlock the potential of their data."
In summation, understanding SPSS is crucial for professionals in various fields, facilitating informed decision-making backed by thorough analysis. A comprehensive grasp of its capabilities enables users to leverage this powerful tool effectively.