Understanding Data Quality: Its Importance and Impact
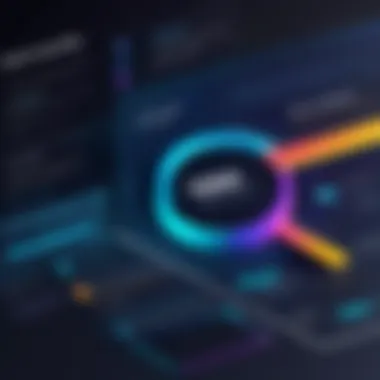
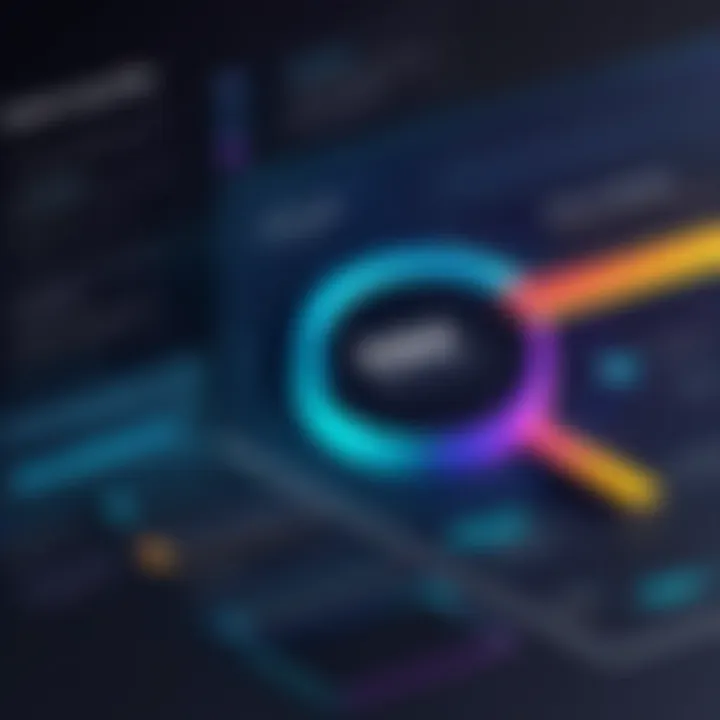
Intro
Data quality is a central concern in this era when decisions are predominantly driven by data. High-quality data enhances decision-making processes and leads to better outcomes. Organizations across sectors rely on data to inform their strategies, assess risks, and drive innovation. However, it seems that poor data quality is often overlooked. It can lead to misguided strategies and costly errors.
Understanding what data quality means is crucial. Data quality involves several dimensions, including accuracy, completeness, consistency, and timeliness. Each of these aspects contributes to the overall integrity and usability of the data. In this article, we will explore these definitions and dimensions. We will also examine the importance and impact of data quality in various contexts. From enhancing decision-making processes to maintaining data integrity, we will cover it all.
The discussion also encompasses technical and managerial considerations necessary for upholding high data quality standards. Numerous strategies exist that organizations can adopt. Identify and mitigate data quality issues proactively to ensure reliable decision-making.
This comprehensive guide aims to educate tech-savvy individuals, business professionals, and IT experts. Let’s dive into the world of data quality and uncover its critical influence on organizational success.
Defining Data Quality
Understanding data quality is foundational to navigating the contemporary landscape of information management. Data quality encompasses a range of attributes that determine the utility and dependability of data. This section will explore crucial elements associated with data quality, emphasizing its role in effective decision-making and operational efficiency.
Basic Concepts and Terminology
Data quality refers to the overall utility of a dataset for its intended uses. Key terms often associated with data quality include accuracy, completeness, consistency, timeliness, and relevance.
- Accuracy: Measures how closely data values match the true values.
- Completeness: Assesses whether all required data is present.
- Consistency: Ensures that data across various databases or systems does not conflict.
- Timeliness: Evaluates whether data is available when needed.
- Relevance: Examines if the data meets the current needs for analysis or decision making.
Each of these elements contributes to an overall assessment of data quality, influencing how effectively information can be utilized in strategic decision-making processes.
Historical Context
The concept of data quality has evolved significantly over the last few decades. Initially, data management focused primarily on data entry and storage, overlooking the importance of quality. As organizations began to rely heavily on data analytics, it became clear that poor data quality resulted in wasted resources and flawed insights.
In the 1980s and 1990s, businesses started to recognize the importance of data integrity. Efforts such as the Total Quality Management movement found their way into data management practices. Reliability standards were introduced to improve the data lifecycle, addressing how data was collected, maintained, and analyzed.
Fast forward to the present, and organizations now leverage sophisticated technologies and methodologies to ensure data quality. Data governance frameworks guide today's best practices, emphasizing the significance of maintaining high-quality data.
"In a data-driven world, understanding and implementing data quality is not just beneficial; it is essential."
The Dimensions of Data Quality
In the realm of data management, understanding the dimensions of data quality is essential for effectively harnessing data's potential. Each dimension represents a critical aspect of data assessment and directly impacts how data is interpreted and utilized. Emphasizing these dimensions allows organizations to identify deficiencies in their data and implement strategies for improvement, thereby enhancing overall decision-making processes.
Accuracy
Accuracy refers to the degree to which data correctly represents the real-world scenarios it is intended to reflect. High accuracy in data is vital because decisions based on inaccurate data can lead to erroneous conclusions and outcomes.
- Factors leading to inaccuracies often include human error, faulty data entry processes, and obsolete information.
- To ensure data accuracy, regular updates and validations are necessary. The implementation of automated data validation tools can also reduce the likelihood of error and improve overall data integrity.
Maintaining accuracy protects an organization’s integrity and credibility by ensuring the decisions made are based on factual information.
Completeness
Completeness assesses whether the full range of necessary data is available for analysis. Missing data, whether from incomplete records or inadequate data source integration, can significantly affect the comprehensiveness of insights derived from data.
For instance, when analyzing customer behaviors, incomplete records might result in an inaccurate portrayal of customer preferences. Ensuring completeness involves:
- Comprehensive data collection strategies,
- Frequent checks for missing values,
- Integration of various data sources that can enhance the overall dataset.
A complete dataset enables organizations to make informed decisions, enhancing operational effectiveness.
Consistency
Consistency in data ensures that the information remains uniform across various datasets and applications. Inconsistent data may arise from discrepancies in data entry practices, resulting in different representations of the same information in multiple systems.
To maintain consistency, organizations should adopt:
- Standardized data entry procedures,
- Regular data reconciliation processes,
- Robust data management frameworks.
These practices help ensure that all teams and departments within an organization are working with the same data, thereby promoting coherent decision-making and interdepartmental alignment.
Timeliness
Timeliness evaluates how current and readily available data is for decision-making. Data that is outdated or not provided promptly can lead to missed business opportunities and ineffective strategies. In fast-paced environments, decision-makers rely on timely data to respond effectively to market changes.
Strategies to enhance timeliness include:
- Real-time data monitoring systems,
- Establishing a timely data update protocol,
- Fostering a responsive data management culture.
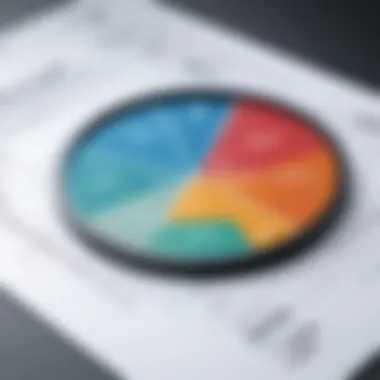
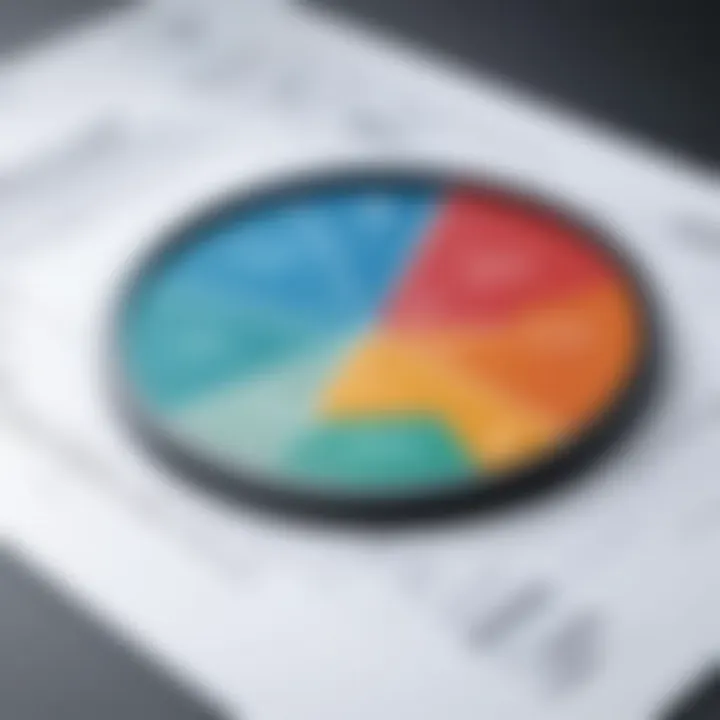
Ensuring timely access to data supports agility in operations and the capacity for proactive decision-making.
Relevance
Relevance pertains to the degree to which data meets the specific needs of the user or application. Even the most accurate data can become extraneous if it is not aligned with the current goals or needs of the organization.
To enhance relevance, organizations should:
- Regularly assess data against user needs,
- Tailor data collection efforts to match key business questions,
- Educate staff on identifying and accessing the most relevant datasets.
By prioritizing relevance, businesses can enhance operational effectiveness and ensure their strategic initiatives are well-informed.
Maintaining these dimensions of data quality is not merely a technical challenge but a strategic imperative that underpins successful decision-making and operational success.
Why Data Quality Matters
Data quality is a crucial aspect of information management that often does not receive the attention it deserves. As organizations continue to accumulate vast amounts of data, the importance of data quality becomes increasingly evident. High-quality data is essential not only for making informed decisions but also for maintaining efficient operations and fostering customer trust. This section will explore the significance of data quality in decision-making, business performance, and customer relationships.
Impact on Decision-Making
Quality data provides a foundation for effective decision-making. Leaders rely on data-driven insights to steer their organizations in the right direction. When data is accurate and complete, decision-makers can analyze trends, forecast outcomes, and execute strategies based on reliable information.
Conversely, poor data quality can lead to misguided choices, resulting in financial losses and reputational damage. In industries where rapid responses are vital, such as finance or healthcare, decisions based on flawed data can have severe consequences. Thus, ensuring data quality is not merely a technical requirement, but a strategic imperative that impacts the very core of an organization’s operations.
Implications for Business Performance
Data quality directly correlates with overall business performance. Organizations that prioritize data integrity tend to experience heightened operational efficiency and improved financial outcomes. When business processes rely on erroneous or incomplete data, inefficiencies arise, ultimately hindering productivity.
A few key factors illustrate this connection:
- Enhanced Productivity: Reliable data streamlines workflows, allowing employees to focus on critical tasks rather than correcting errors.
- Cost Reduction: Investing in high data quality may prevent costly mistakes, such as inaccuracies in billing or misguided marketing efforts.
- Competitive Advantage: Organizations that harness quality data gain insights into market trends and customer preferences faster than their competitors.
Prioritizing data quality is therefore an investment, leading to long-term benefits in productivity and profitability.
Customer Trust and Reputation
In today’s hyper-connected marketplace, customers are more informed than ever. They expect transparency and accuracy from the brands they engage with. High-quality data fosters customer trust by ensuring that communications, product offerings, and services accurately reflect reality.
Moreover, poor data can lead to significant relationship breakdowns. For instance, when a business fails to provide timely and accurate information about order status or product availability, it risks losing customers. Trust, once lost, is incredibly hard to regain. Organizations need to recognize that their reputation is intertwined with data quality. As such, maintaining integrity in data is not only beneficial for decision-making and performance but also vital for nurturing lasting customer relationships.
"Good data is like good currency; it holds value and can be trusted to serve your best interests."
Consequences of Poor Data Quality
In the realm of data management, the consequences of poor data quality are often severe and far-reaching. It is not merely a technical issue, but a challenge that can alter strategic outcomes, impact customer relationships, and even threaten organizational reputation. Understanding the various dimensions of these consequences is essential for any business aiming to leverage data as a key resource in decision-making and operational efficiency.
"Quality is not an act, it is a habit." – Aristotle
Financial Costs
Organizations that underestimate the impact of poor data quality can face significant financial repercussions. This can manifest in several ways, including wasted investments in ineffective marketing campaigns, incorrect pricing strategies, or misallocation of resources. The infamous study by IBM estimates that businesses lose approximately $3.1 trillion a year due to poor data quality.
- Direct Costs: These involve expenses resulting directly from errors, such as correcting inaccurate data entries or dealing with the fallout from failed projects. For instance, if a company misquotes its inventory levels due to faulty data, it may end up overstocked or understocked, leading to lost sales opportunities.
- Indirect Costs: These costs are less visible but equally detrimental. Poor data can lead to bad business decisions, which are often irreversible. A survey from Gartner highlights that 70% of business leaders attribute their company's failure to ineffective data management. The cumulative effect of these poor decisions could severely impact the bottom line and erode profit margins.
Operational Inefficiencies
Another significant consequence of poor data quality is the emergence of operational inefficiencies. When data is inaccurate, inconsistent, or incomplete, it complicates various processes within an organization.
- Time Wasted: Employees may spend excessive time validating or correcting data rather than focusing on core business activities. For example, sales teams may experience delays when chasing down customer information due to inaccuracies, leading to missed opportunities and diminished productivity.
- Impact on Systems: Poor data quality can hinder the performance of IT systems, resulting in increased downtime and maintenance costs. If critical data driving operational processes is flawed, it can lead to cascading failures across departments.
Legal and Compliance Risks
Organizations face serious legal and compliance implications from inadequate data quality. Many industries are subject to rigorous data protection laws and regulations. A breach due to poor data management can lead to severe penalties.
- Regulatory Violations: Regulatory bodies, such as the General Data Protection Regulation (GDPR) in Europe, impose strict guidelines on data management practices. Non-compliance can result in hefty fines and damage to reputation. Companies could find themselves entangled in lengthy legal battles that distract from their core mission.
- Litigation Risks: In the event of data mismanagement that affects customers or clients, companies may face lawsuits. For instance, inaccurate financial reporting could mislead investors, resulting in legal claims against executives. Such scenarios not only harm the organization’s finances but also diminish trust among stakeholders.
In summary, it is clear that poor data quality has severe consequences that organizations cannot afford to ignore. The financial costs, operational inefficiencies, and legal repercussions create a compelling case for prioritizing data integrity and implementing robust data quality management practices.
Promoting High Data Quality Standards
Promoting high data quality standards is imperative in a world increasingly reliant on data-driven decision-making. Organizations must implement effective practices in order to cultivate a culture that prioritizes data integrity and reliability. A commitment to data quality not only impacts internal processes but also influences customer satisfaction and market competitiveness.
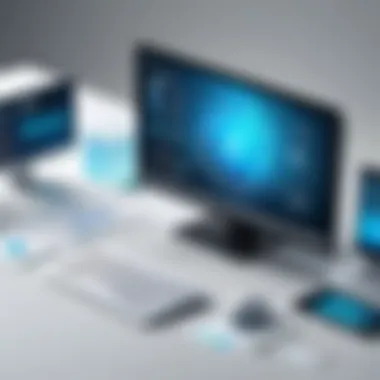
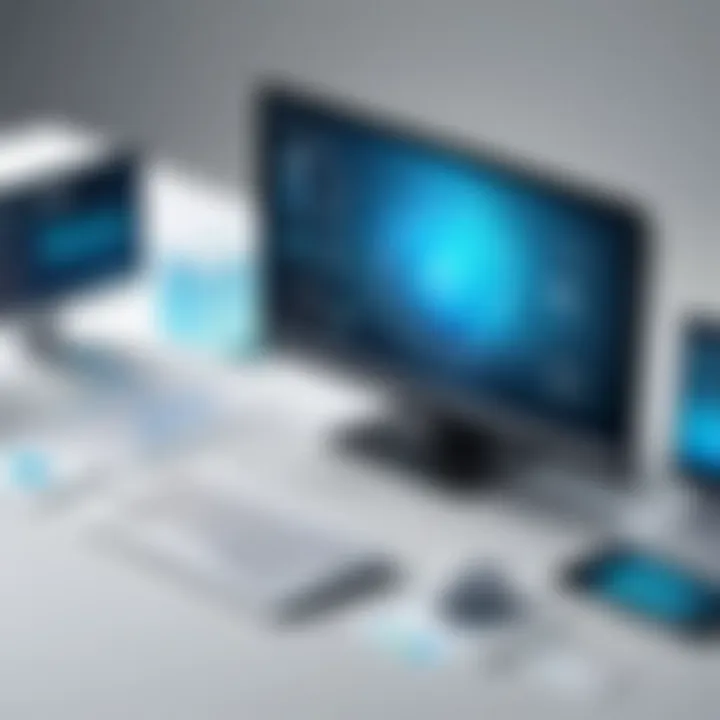
Data quality standards help to define what constitutes accurate, complete, and timely data. By identifying and adhering to these standards, businesses can minimize risks associated with poor data quality, such as financial losses and damaged reputations. Stakeholders across the board, from executives to data analysts, benefit from reliable data that supports sound decision-making.
Data Governance Frameworks
A robust data governance framework is a foundational pillar for maintaining high data quality standards. This framework outlines the policies, procedures, and roles necessary to ensure that data is managed properly across the organization. Effective data governance involves identifying data owners, establishing data stewardship roles, and creating guidelines for data handling.
Organizations should consider these elements when developing a data governance framework:
- Data Accuracy: Establishing responsibility for data accuracy is essential. Clear assignments can mitigate errors.
- Data Access: Setting rules around who can access data ensures that sensitive information remains secure while promoting collaboration among authorized personnel.
- Compliance: Adhering to legal and regulatory mandates (such as GDPR or HIPAA) requires ongoing assessment of data management practices.
Implementing such frameworks not only ensures accountability but also nurtures a shared understanding of data’s value within the company.
Data Quality Tools and Technologies
The right tools and technologies can significantly enhance the ability to promote high standards of data quality. There are various software solutions that can automate data quality checks, monitor data anomalies, and facilitate data cleansing. Commonly used tools include Informatica, Talend, and Microsoft Azure Data Factory.
When selecting data quality tools, organizations should consider:
- Functionality: Does the tool cover necessary features like profiling, cleansing, and enrichment?
- Integration: How well does it integrate with existing systems? Seamless integration can streamline operations.
- User experience: An intuitive interface can enhance adoption and usage among staff.
Using such technologies not only simplifies day-to-day data management tasks but also empowers teams to focus on more strategic initiatives.
Staff Training and Awareness
No matter how robust the tools or frameworks are, the human element remains crucial. Training staff effectively on data handling practices significantly contributes to data quality. Awareness programs can emphasize the importance of data accuracy, completeness, and timeliness. Employees should understand their roles in maintaining data quality and how their decisions impact overall business performance.
Programs can include:
- Workshops: Regular workshops encourage hands-on practice with data tools and reinforce data governance protocols.
- E-learning Modules: These can allow employees to learn at their own pace about data management best practices.
- Feedback Mechanisms: Establishing channels for feedback helps to address data quality issues promptly and fosters continuous improvement.
Ultimately, investing in staff training leads to a more informed workforce, better equipped to uphold data quality standards.
Challenges in Maintaining Data Quality
In the realm of data quality, understanding the challenges is crucial. These obstacles can undermine the effort to achieve high standards and affect the decision-making processes within organizations. Recognizing and addressing these challenges helps businesses maintain their competitive edge in a data-driven world. Each aspect plays a significant role in the overall quality of data, influencing how it is utilized and managed.
Data Silos and Fragmentation
Data silos refer to isolated pockets of data that are not easily accessible across an organization. This fragmentation occurs often due to poor communication between departments and the use of different systems. When data is siloed, it becomes difficult to consolidate and analyze. Inaccurate or obsolete information can enter the decision-making process, leading to flawed strategies.
Mitigating the effects of data silos requires collaboration among teams. Organizations must implement integrated systems and shared platforms to ensure seamless access to data. By encouraging a culture of sharing and transparency, businesses can enhance their data quality and make informed decisions based on comprehensive insights.
Rapidly Changing Data Environments
The digital landscape is in a state of constant flux. Data sources multiply rapidly, and the nature of data evolves with trends and user behavior. Maintaining data quality in such an environment is challenging. Organizations struggle to keep up with data updates and changes in structure. Without rigorous data management strategies, outdated or incorrect data might be utilized in operations.
To combat this issue, regular reviews and adjustments of data processes are key. Companies should invest in agile data management solutions that can adapt to changing conditions. Emphasizing flexibility allows organizations to respond to new data sources, enhancing overall quality and usability of data over time.
Integration Issues Across Systems
Integration across various systems often presents a hurdle for data quality management. Organizations utilize a handful of software solutions, each creating distinct data formats. Merging these disparate data streams can generate conflicts and discrepancies. This lack of standardization exposes data to risks such as errors and duplications.
To tackle the integration challenge, businesses should prioritize the use of data integration tools. These tools can help reconcile differences between systems, ensuring data remains consistent and valid across platforms. Effective integration strategies not only improve quality but also streamline business processes, enhancing efficiency and productivity.
"Data quality management can determine the success or failure of strategic initiatives across industries."
In summary, addressing the challenges in maintaining data quality is essential for businesses aiming to leverage their data fully. By focusing on the issues of data silos, changing environments, and integration problems, organizations can create a robust data management framework. This leads to improved data integrity and ultimately, better decision-making capabilities.
Measuring Data Quality
Measuring data quality is a fundamental step in ensuring that the data used for analysis and decision-making is reliable and useful. It serves as a benchmark for assessing the integrity of data across various processes. As organizations become increasingly data-driven, the ability to measure quality becomes not only valuable but essential. Data quality metrics enable companies to pinpoint issues, monitor deficiencies, and implement improvements systematically.
Different aspects contribute to measuring data quality. Among these, the establishment of quality metrics and key performance indicators (KPIs) plays a crucial role. Organizations need measurable and quantifiable standards to determine how well their data meets established criteria. The clarity brought by specific metrics makes it easier to articulate data quality issues and address them effectively.
Furthermore, conducting regular audits and assessments is imperative. These processes provide insights into ongoing data management practices, allowing organizations to identify trends and recurring problems. Therefore, continuous monitoring promotes sustained data integrity and ensures it remains aligned with the strategic goals of the organization.
"Consistent measurement of data quality transforms data into a strategic asset, supporting informed decision-making and enhancing operational efficiencies."
Quality Metrics and KPIs
Quality metrics serve as specific standards for evaluating the data's effectiveness. Metrics such as accuracy, completeness, consistency, and timeliness help organizations understand where their data stands in relation to desired quality levels. Each of these metrics comes with particular techniques for measurement.
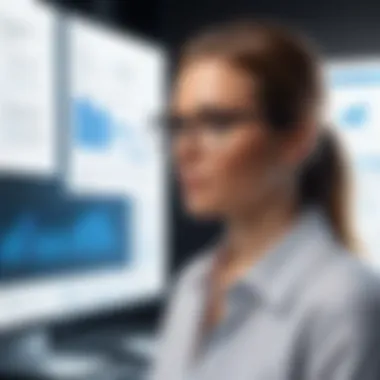
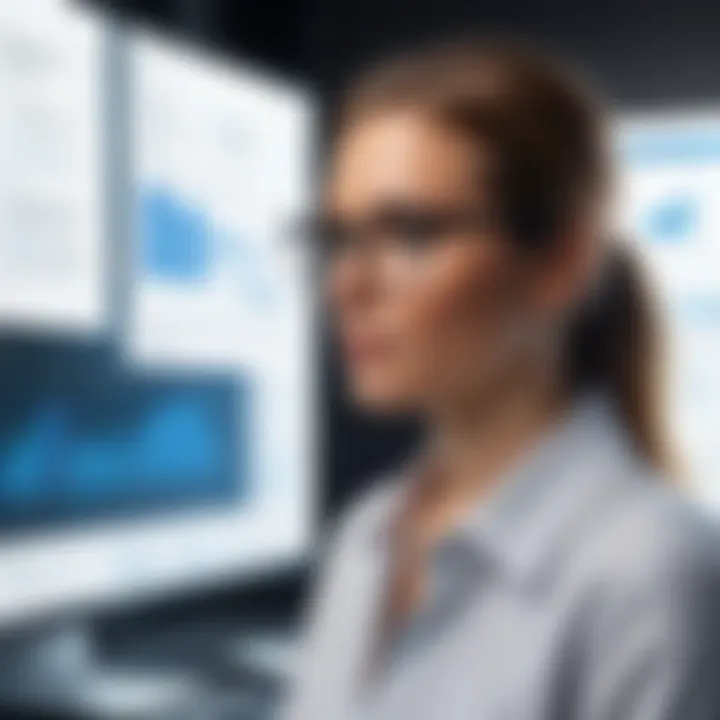
- Accuracy refers to the closeness of data to its true value. Metrics can be implemented to measure the error rates and identify inaccuracies.
- Completeness looks at whether all necessary data is present. Assessing this often involves creating lists of required fields and checking for missing entries.
- Consistency gauges whether data across various datasets holds the same values. A common method includes matching records from different sources to see if discrepancies exist.
- Timeliness emphasizes the data’s availability when needed. Analysis can include time lags between data generation and reporting.
To gauge how these metrics perform, KPIs such as data entry error rates and user satisfaction scores can be established. Setting benchmarks allows organizations to track improvements or declines over time. This data-driven approach is essential for making informed decisions regarding data quality management.
Regular Audits and Assessments
Conducting regular audits and assessments forms a vital component of maintaining high data quality standards. Audits ideally should occur as part of a scheduled routine but can also be instigated by significant organizational changes.
Through thorough audits, organizations can:
- Identify quality gaps in current data.
- Verify compliance with established data governance policies.
- Uncover adverse trends that could affect operational performance.
Assessments can include both qualitative and quantitative evaluations of data. Including feedback loops where stakeholders provide insights on data usability will uncover subjective issues not represented in quantitative metrics. Hence, organizations can adjust their strategies based on practical usability concerns.
In summary, measuring data quality via engaging metrics and conducting audits forms a crucial part of effective data management. By adopting these practices, organizations can enhance decision-making processes and ensure the integrity of their data throughout different applications.
Data Quality in Different Industries
In an era heavily dictated by data, the significance of quality data cannot be overstated. Each industry shapes its data quality requirements based on its unique operational needs. The implications of poor data quality can vary, but they invariably lead to financial losses, inefficiencies, and reputational damage. This section delves into the importance of data quality across various sectors, highlighting specific elements, benefits, and considerations.
IT and Software Development
The IT and software development industry relies fundamentally on data to drive innovation and efficiency. High-quality data is crucial for software developers to create applications that meet user demands effectively. Quality data enables developers to analyze user behavior accurately, forecast application trends, and understand system performance metrics.
Key benefits of data quality in this domain include:
- Improved software testing: Accurate data helps in robust testing processes, reducing the number of bugs and enhancing user experience.
- Effective project management: Reliable data aids in resource allocation and tracking project progress systematically.
- Enhanced analytics: Good data informs better decision-making in upgrading software features and overall project direction.
Healthcare
In healthcare, data quality is paramount for patient safety and regulatory compliance. High-quality data can influence clinical decisions and treatment outcomes, making it essential for healthcare providers to maintain accurate and complete patient records.
Some critical considerations include:
- Patient outcomes: Reliable data can help in improving patient management and outcomes through evidence-based decisions.
- Operational efficiency: Quality data drives operational decisions, improving scheduling, staffing, and resource allocation.
- Compliance requirements: Healthcare data is subject to strict regulations; maintaining data integrity is vital to meet these obligations.
Finance and Banking
In the finance and banking sector, data quality is crucial due to heavy regulatory scrutiny and the inherent risks associated with financial transactions. Accurate financial data reduces the likelihood of errors that can lead to significant monetary losses and compliance issues.
The key elements of data quality in finance include:
- Risk management: High-quality data helps in identifying and mitigating financial risks, leading to more stable operations.
- Regulatory compliance: Financial institutions must adhere to regulations that require precise reporting; poor data can result in severe penalties.
- Customer trust: Accurate data fosters customer loyalty, as clients value reliable financial services.
Retail and E-commerce
In retail and e-commerce, the dynamics of consumer behavior underscore the importance of data quality. Retailers rely on accurate and timely data to drive inventory management, marketing efforts, and customer relationship strategies.
Addressing data quality in this industry brings forth several benefits:
- Enhanced customer experience: Accurate product information and personalized offers improve customer satisfaction and retention.
- Inventory management: Quality data supports effective tracking and management of stock levels, preventing both overstock and stockouts.
- Marketing effectiveness: Reliable data on consumer preferences aids in targeted marketing, maximizing the return on investment.
Maintaining high data quality standards across these industries is not merely advantageous; it is essential for sustaining competitive advantage in today's data-driven landscape.
Future Trends in Data Quality Management
As organizations increasingly rely on data for strategic decisions, understanding future trends in data quality management becomes essential. The landscape of data is ever-changing, driven by advancements in technology and evolving business needs. Looking ahead, it is crucial to embrace these trends to ensure data remains both reliable and actionable. Future trends can provide distinct benefits while highlighting key considerations for effective data governance.
Artificial Intelligence and Machine Learning
Artificial Intelligence (AI) and Machine Learning (ML) are revolutionizing data quality management. These technologies can automate routine data quality checks, significantly reducing the manual effort required.
By analyzing patterns in data, machine learning algorithms can identify anomalies that might indicate poor data quality. For example, when a dataset shows unexpected inconsistencies, AI tools can alert users to investigate further. This not only enhances efficiency but also improves accuracy over time as the system learns from previous data quality issues.
Moreover, organizations can take advantage of predictive analytics. By anticipating potential data quality problems before they occur, businesses can take proactive measures to maintain high standards. This foresight minimizes disruptions and supports smoother operations in the long run.
Automated Data Quality Tools
The rise of automated data quality tools is another significant trend. These tools provide comprehensive solutions for cleansing, validating, and enhancing data integrity without extensive human intervention. Businesses can implement systems like Talend, Informatica, or Apache NiFi, which streamline data processes and reduce errors.
Key benefits of these tools include:
- Scalability: They can handle large volumes of data efficiently, making them ideal for growing organizations.
- Consistency: Automated processes ensure that data is handled uniformly, reducing the risk of human error.
- Time Savings: Automation takes over labor-intensive tasks, allowing teams to focus on strategic initiatives rather than routine data management.
"Investing in automated data quality tools is investing in the future of your organization."
Data quality management is becoming more sophisticated. As technology continues to evolve, embracing these trends can put organizations at a competitive advantage. The integration of AI, ML, and automation will likely reshape the landscape, allowing organizations to maintain accurate, relevant, and timely data, thereby supporting overall business objectives effectively.