Harnessing Predictive Analytics with SAP Analytics Cloud
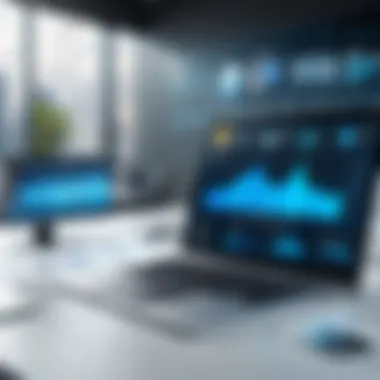
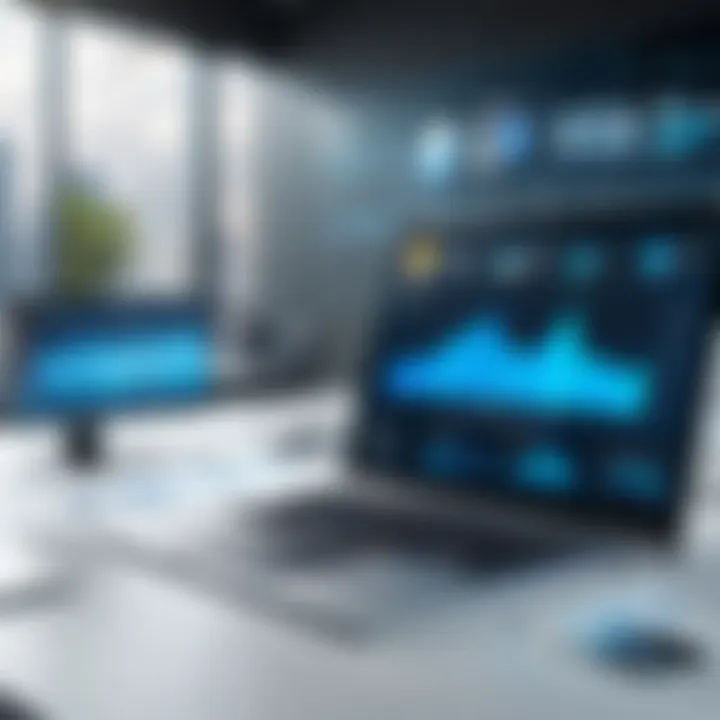
Intro
In today's data-driven world, harnessing the power of analytics is no longer a luxury but a necessity for businesses aiming for growth and operational efficiency. SAP Analytics Cloud stands out as a premier tool that allows organizations to dive deep into predictive analytics. This capability enables them to forecast trends, make informed decisions, and streamline operations effectively. Through this article, we’ll peel back the layers on what makes SAP Analytics Cloud a robust option for leveraging predictive analytics.
Software Overview and Benefits
SAP Analytics Cloud is a comprehensive platform that integrates business intelligence, augmented analytics, and predictive capabilities into one user-friendly interface. At its core, the software facilitates data exploration, visualization, and reporting, all tied together with predictive modeling functionalities. Some notable features include:
- Predictive Scenario Planning: Allows businesses to simulate various scenarios and understand potential outcomes based on changing variables.
- Smart Insights: Generates automatic insights from data without requiring deep analytical knowledge.
- Collaboration Tools: Teams can share dashboards and reports, fostering a culture of data-driven decision-making.
The benefits of utilizing SAP Analytics Cloud are manifold. Firstly, it helps in addressing specific needs through its customizable dashboards, thereby making it easier for users to access the information most relevant to them. Additionally, companies can expect improved operational efficiency through faster data analyses and timely insights. This ultimately leads to making educated decisions that can positively impact the bottom line.
Pricing and Plans
Regarding budget considerations, SAP Analytics Cloud offers multiple subscription plans that cater to the varying needs of businesses. The pricing is structured based on a per-user basis, allowing firms to scale as they grow. Currently, enterprise plans may start around $21 per user per month, with different functionalities depending on the tier. It’s advisable to compare this with competitors, such as Tableau or Microsoft Power BI, which also offer analytics solutions at competitive prices but might lack certain advanced predictive capabilities available in SAP Analytics Cloud.
Performance and User Experience
When it comes to performance, SAP Analytics Cloud is highly regarded for its speed and reliability. Users report efficient processing even with large datasets, ensuring minimal delay in generating insights. The interface is designed with the user in mind, making it intuitive for individuals who may not possess significant analytical expertise.
User feedback often highlights that the learning curve is manageable, particularly because the platform offers guided tutorials and interactive features. Some users may encounter issues with data loading times, but these are typically minor in comparison to the overall experience.
Integrations and Compatibility
The true strength of SAP Analytics Cloud lies in its ability to integrate seamlessly with a myriad of other tools and platforms. It can connect with popular databases, including SAP HANA, Salesforce, and even non-SAP products like Google Analytics. This makes it a flexible option for businesses that rely on diverse systems for their operations.
Moreover, the software is compatible with various operating systems. Whether you’re on Windows, macOS, or even accessing through mobile devices, the application has adapted well to maintain functionality.
Support and Resources
To ensure users get the most out of SAP Analytics Cloud, several support options are available. Organizations can access customer support through dedicated channels, including chat and email. Also, SAP provides a wealth of resources such as comprehensive guides, detailed tutorials, and a user community for peer support.
For further learning, users can find additional materials that cover advanced features and best practices. These resources enhance the overall user experience and facilitate a deeper understanding of the software’s capabilities, ultimately leading to better data-driven decisions in their respective organizations.
By integrating advanced predictive analytics through SAP Analytics Cloud, businesses can truly transform their decision-making processes, unlocking opportunities they may have previously overlooked.
Prelims to SAP Analytics Cloud
The shift towards data-driven decision-making has transformed the landscape of business intelligence. In this context, SAP Analytics Cloud plays a pivotal role in leveraging predictive analytics. This powerful tool equips organizations with the ability to analyze historical data, draw insights, and forecast future trends. By understanding the capabilities of SAP Analytics Cloud, businesses position themselves to unlock value from their datasets, making informed decisions that drive performance and growth.
Overview of SAP Analytics Cloud
SAP Analytics Cloud is a unified SaaS (Software as a Service) solution that combines business intelligence, augmented analytics, and planning capabilities. It caters to organizations seeking a seamless approach to data integration and visualization. One standout feature is how it integrates with various SAP and non-SAP data sources, offering flexibility and scalability.
The platform emphasizes user-friendly interfaces, allowing users of varying tech-savvy levels to explore data without getting lost in complexities. Dashboards are customizable, enabling stakeholders to see the metrics that matter most to them. The underlying architecture is geared towards real-time analytics, making it an ideal fit for organizations aiming to be agile in their operations. In essence, SAP Analytics Cloud is not just another analytics tool; it’s a gateway to a profound understanding of business dynamics.
Importance of Data Analytics in Business
Data analytics has become a cornerstone in the strategic framework of modern businesses. Through it, companies can uncover insights that might otherwise remain hidden. The importance of data analytics mangments several fronts:
- Informed Decision-Making: Combining historical data with advanced analytics fosters better decisions. Organizations can identify patterns, trends, and anomalies to guide their strategies.
- Competitive Advantage: Companies that effectively leverage data analytics can outpace their competitors by adapting quickly to market changes or consumer preferences. This adaptability can translate into increased market share.
- Operational Efficiency: Analytics pinpoints inefficiencies in processes, leading to streamlined operations. When businesses know where to improve, they can cut costs and enhance performance.
"In today’s fast-paced business world, checking the pulse of your data is not optional—it’s essential to survival."
In summary, the integration of data analytics within business processes is not merely beneficial; it is critical. With solutions like SAP Analytics Cloud, organizations are not just participating in the analytics revolution—they are at the forefront, reaping the rewards.
Understanding Predictive Analytics
Predictive analytics emerges as an essential element in the landscape of modern business intelligence. It provides an avenue for organizations to harness existing data with the aim of predicting future outcomes. This section delves into the nuts and bolts of predictive analytics, elucidating its significance and how it manifests within the capabilities of SAP Analytics Cloud.
Definition and Key Concepts
At its core, predictive analytics refers to techniques that leverage statistical algorithms and machine learning approaches to identify the likelihood of future outcomes based on historical data. This process typically involves several steps, including data aggregation, data mining, and predictive modeling. The primary goal here is not just to examine what has happened, but to forecast what could happen next.
Several key concepts characterize predictive analytics:
- Data Mining: This involves discovering patterns and relationships within large sets of data, facilitating more informed predictions.
- Statistical Modeling: Techniques such as regression analysis or time series forecasting are prevalent here. They allow businesses to understand relationships between variables.
- Machine Learning: Algorithms that improve automatically through experience can adjust predictions as they process new data.
Understanding these concepts is vital for professionals who aim to utilize SAP Analytics Cloud effectively in their predictive endeavors.
The Role of Predictive Analytics in Decision-Making
Decision-making is a pivotal aspect of any business operation. Here, predictive analytics plays a transformative role by providing data-driven insights that can significantly enhance the quality of decisions made.
When organizations leverage predictive analytics, they empower themselves to:
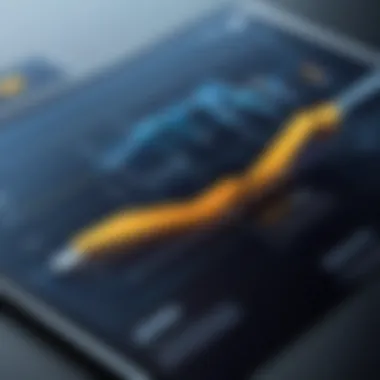
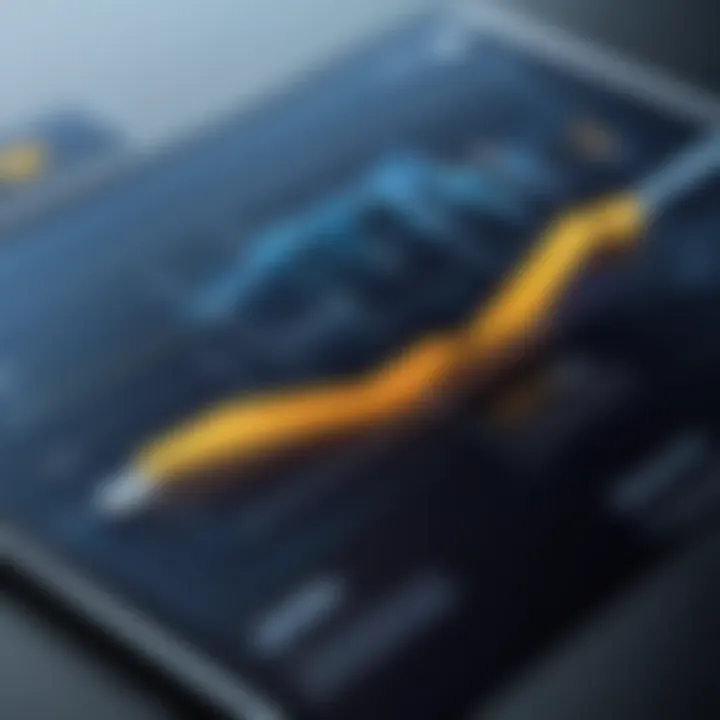
- Anticipate market trends and shifts, allowing for proactive rather than reactive measures.
- Optimize resource allocation by forecasting demand, leading to better inventory management in sectors like retail.
- Mitigate risks by identifying potential issues before they manifest, fostering agility in operations.
"In today’s fast-paced business environment, organizations utilizing predictive analytics are often one step ahead, allowing them to navigate uncertainties with greater assurance."
Ultimately, the integration of predictive analytics fosters a culture of informed decision-making. Not only does it open up potential avenues for efficiency and profitability, but it also arms businesses with the insights necessary to stay ahead of competition.
As SAP Analytics Cloud continues to evolve, understanding the foundations of predictive analytics becomes all the more critical for organizations aiming to thrive in an increasingly data-centric world.
Key Features of SAP Analytics Cloud
Understanding the key features of SAP Analytics Cloud is essential for organizations looking to enhance their predictive analytics capabilities. These features directly influence how businesses can utilize data to make more informed decisions, identify trends, and optimize processes. They are not just bells and whistles; they’re core functionalities that align with the need for efficiency and insight in a data-driven world. Let’s dive into the specifics of what makes SAP Analytics Cloud stand out in the competitive landscape of analytics solutions.
Data Connectivity and Integration
One of the principal features of SAP Analytics Cloud is its robust data connectivity and integration capabilities. This platform supports a broad range of data sources, including on-premises systems, cloud databases, and third-party applications. Organizations can seamlessly connect their data sources - be it SAP HANA, Microsoft SQL Server, or even Google Sheets. This flexibility allows for a unified view of data, breaking down silos that often hinder analytics efforts.
Moreover, the platform supports real-time data integration. This means that businesses no longer have to wait for end-of-day reports to gain insights. Instead, they can react promptly to unexpected shifts in data trends, which is crucial in today’s fast-paced environment.
In practical terms, extracting data from disparate sources and consolidating it into one comprehensive platform leads to better analysis and more actionable insights. This lowers the barrier to entry for small and medium-sized businesses that may lack extensive IT infrastructures, making advanced analytics more accessible than ever.
User Interface and Experience
The user interface (UI) of SAP Analytics Cloud is designed with usability in mind. An intuitive and clean interface allows both technically inclined users and more casual data explorers to navigate the tool effectively. This matters in an organization where not everyone is a data specialist; making insights accessible to a broader audience is key.
Another aspect worthy of mention is the customization options available. Users can tailor dashboards and reports to focus on metrics and visuals that are most relevant to their specific needs. Having a personalized experience enhances user engagement and encourages teams to utilize these analytical tools regularly, fostering a data-driven culture throughout the organization.
Additionally, responsiveness across devices allows users to access data on desktop or mobile, making it feasible to monitor KPIs and trends on the go. This kind of flexibility is not just convenient; it equips decision-makers with the tools they need to act quickly when opportunities or issues arise.
Collaboration and Sharing Tools
Collaboration features in SAP Analytics Cloud are another standout element. In an age where teamwork is critical, this platform facilitates real-time collaboration across departments. Teams can work together on dashboards, share insights, and even annotate reports—creating a feedback loop that fosters continuous improvement.
Furthermore, sharing tools allow users to disseminate insights widely without cumbersome processes. They can easily export reports or share links to dashboards directly with stakeholders. This ensures that the right information reaches the right people in a timely manner. The ease of sharing mitigates the chances of miscommunication, which is a frequent obstacle in organizational decision-making.
"Collaboration in analytics has become indispensable. It’s not just about finding insights, but also about sharing and discussing them in real time."
In sum, the features of SAP Analytics Cloud are finely aligned with modern business needs. Data connectivity ensures comprehensive integration of information. The user interface promotes ease of use for all skill levels, and collaboration tools pave the way for seamless teamwork. Together, these elements empower organizations to leverage predictive analytics efficiently, driving informed decision-making and enhancing overall performance.
The Predictive Analytics Process
The predictive analytics process is a cornerstone in the journey toward data-driven decision-making, especially when harnessed through SAP Analytics Cloud. To put it simply, this process is not just a series of steps; rather, it is more like a roadmap that guides businesses from raw data to actionable insights. With the explosion of data in recent years, distinguishing valuable insights from noise is crucial. The predictive analytics process ensures organizations can effectively sift through this vast information and make informed strategic decisions.
Understanding this process involves recognizing its three main components: data preparation and cleansing, model creation and validation, and deployment and monitoring. Each of these elements contributes significantly to the overall effectiveness of predictive analytics.
Data Preparation and Cleansing
Data preparation and cleansing is the first critical step in the predictive analytics process. This phase can often be the difference between a successful project or one that never gets off the ground. To get good results from analytics, it’s essential to start with good data. Raw data may come from various sources, including databases or external APIs, but it is seldom in a usable format.
Cleaning data and preparing it for analysis means identifying and rectifying errors, removing duplicates, and ensuring consistency. Think of it as spring cleaning for data: you sift through, discard the clutter, and organize what you retain. In SAP Analytics Cloud, tools streamline this process substantially, allowing users to drag and drop datasets and define rules for cleansing. Here are some common tasks involved in this phase:
- Eliminating duplicates: Identifying and removing redundant entries to ensure data integrity.
- Handling missing values: Making decisions about how to fill gaps, whether through estimation or exclusion.
- Normalization: Standardizing data formats to promote consistency across the dataset.
This meticulous preparation ultimately lays the foundation for more accurate predictions and better business insights.
Model Creation and Validation
Once the data is prepped and primed, the next step is model creation and validation. In essence, this part is akin to crafting a piece of art: you start with materials (clean data) and then shape it into something coherent and meaningful. In SAP Analytics Cloud, users have access to various algorithms and methodologies that help create predictive models tailored for specific business needs.
Model creation can involve selecting the right algorithms, defining parameters, and training the models using historical data. However, the journey does not end there; it’s also vital to validate these models to ensure they deliver reliable predictions. Validation typically entails:
- Testing: Utilizing a portion of historical data reserved for testing to measure the accuracy of the model.
- Cross-validation: Running multiple tests to evaluate the model's robustness and to avoid overfitting.
- Metrics assessment: Employing various performance metrics such as precision, recall, or F1 score to gauge model effectiveness.
An effective model can significantly enhance forecasting abilities, bestowing businesses with insights that drive strategic actions.
Deployment and Monitoring
The final step in the predictive analytics process is deployment and monitoring, where the rubber meets the road. Once a model has been validated, the next move is putting it into action. In SAP Analytics Cloud, this can often be achieved through easy integrations with existing business applications, aiding seamless transitions.
However, deployment is not a set-it-and-forget-it scenario. Continuous monitoring is key to ensuring that the predictive model remains relevant and effective. This process involves:
- Real-time data feeding: Continuously updating the model with new data to keep predictions fresh and accurate.
- Performance evaluation: Regularly assessing how well the model performs in the real world and making necessary adjustments.
- Feedback loops: Creating mechanisms for users to provide insights that can help fine-tune models over time.
This dynamic approach allows organizations to adaptively utilize their predictive analytics capabilities, enhancing overall operational efficiency and strategic insights.
Effective predictive analytics, supported by a robust process, empowers organizations to not only anticipate future trends but also to adapt and respond proactively to various challenges.
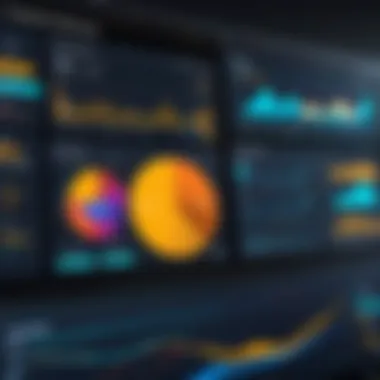
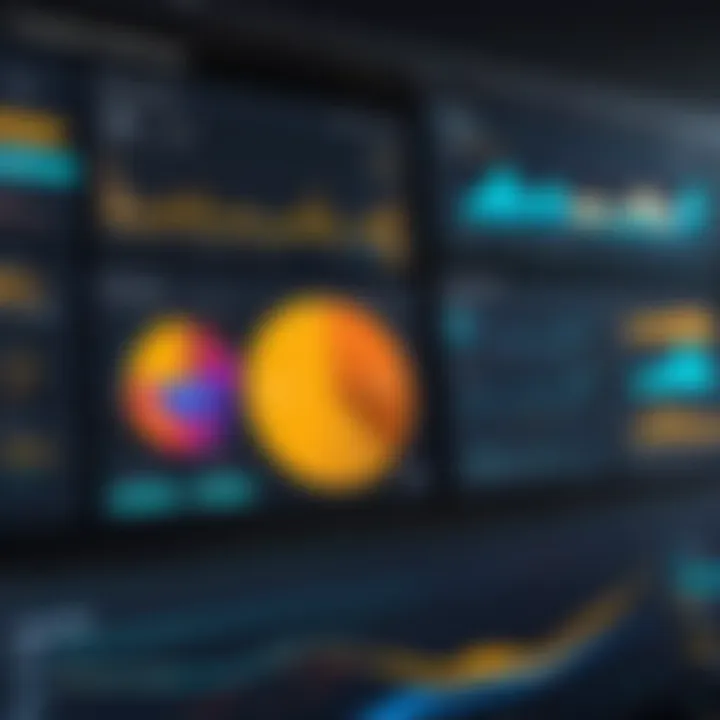
Benefits of Using SAP Analytics Cloud for Predictive Analytics
In an era where data reigns supreme, organizations are scrambling to harness the power of predictive analytics to guide their strategies. SAP Analytics Cloud stands out as a robust platform that amplifies this analytical capability. It's not just about crunching numbers; it's about generating insights that can propel businesses into the future. The benefits of adopting SAP Analytics Cloud for predictive analytics are manifold, ranging from increased accuracy in forecasting to a significant boost in overall business performance.
Enhanced Forecasting Accuracy
With predictive analytics, accuracy is the name of the game. Utilizing SAP Analytics Cloud allows organizations to refine their forecasting methods, making them far more reliable. Through advanced algorithms and machine learning, this platform can sift through vast amounts of historical and real-time data, offering predictions that are grounded in solid analytics rather than gut feeling.
A notable advantage here is the tool's ability to adapt based on changing data patterns. For instance, a retail business can employ this feature to better predict seasonal product demands by analyzing purchase trends from previous years and current market conditions. As the data inputs shift, the forecasts adjust automatically. Hence, organizations aren't left hanging on outdated assumptions which can lead to surplus inventory or disappointing stock-outs.
"The sooner businesses leverage accurate forecasting, the more competitive edge they maintain in unpredictable markets."
Improved Business Performance
Predictive analytics does not only enhance the accuracy of forecasts; it also translates directly into improved business performance. Organizations that utilize SAP Analytics Cloud can make decisions backed by future insights rather than reactive approaches. When businesses embrace data-driven decision-making, their operational efficiencies tend to rise significantly.
For example, in the manufacturing sector, companies can predict machinery maintenance needs before they even arise. By analyzing wear and tear through predictive analytics, firms can minimize downtime and maximally utilize their equipment. This proactive approach streamlines operations and can lead to significant cost savings, turning potential losses into gains, or at least keeping the ship steady.
Streamlined Reporting and Insights
SAP Analytics Cloud doesn't just stop at delivering accurate predictions; it also offers a sleek user interface that simplifies data reporting. In today’s fast-paced business environment, the ability to transform complex data insights into easy-to-understand reports can be a game changer. End-users can craft visually appealing dashboards that present key insights at a glance, making the data accessible to all stakeholders—especially for those who might not be as tech-savvy.
Moreover, organizations can share these insights seamlessly across different departments, creating a unified understanding of business objectives. This collaborative approach is essential for ensuring that all sectors—from sales to finance—are aligned in their strategies. When necessary data is right at one's fingertips, businesses can pivot strategies swiftly, adapting to new opportunities or sudden market shifts.
In sum, the benefits of utilizing SAP Analytics Cloud for predictive analytics go far beyond mere number crunching. It's about equipping businesses with the tools to harness their data for strategic advantage, elevating performance, and ensuring agile, informed decision-making.
Use Cases of Predictive Analytics in Various Industries
Predictive analytics has emerged as a game changer across various sectors. Its impact is especially discernible in how businesses allocate resources, make strategic decisions, and ultimately engage customers. The following sections delve into how different industries harness predictive analytics to stay ahead of the curve, driving efficiency and unlocking value from data.
Retail Industry Applications
In the retail sector, predictive analytics is a vital tool for understanding consumer behavior. Retailers leverage data derived from purchase histories, browsing behaviors, and customer feedback to anticipate trends. For instance, by analyzing shopping habits, a company like Walmart can forecast what products will be in demand for the upcoming holidays.
Companies can use predictive analytics for inventory management as well. By predicting sales trends, retailers can optimize stock levels, reducing excess inventory and minimizing the risk of stockouts. This balance translates into improved cash flow and better shelf space management.
Benefits of predictive analytics in retail include:
- Enhanced customer targeting and personalization strategies.
- Increased operational efficiency through better demand forecasting.
- Improved customer satisfaction as products align closely with consumer needs.
"In retail, it ain't just about having the right product at the right time; it's about knowing your customer better than they know themselves."
Finance and Banking Insights
The finance and banking industries have quickly recognized the potential of predictive analytics. Here, the focus often areas around risk management and enhancing customer experience. Financial institutions can analyze vast amounts of transaction data to identify anomalies indicative of fraud, flagging them before any significant losses occur.
Moreover, banks use predictive models to assess loan applications. By examining factors like credit scores, historical data, and even social media activity, institutions can make more informed lending decisions. This analytical approach results in fewer delinquent loans while also expanding access to credit for lower-risk clients.
Key applications include:
- Fraud detection and prevention, securing transactions.
- Credit risk assessment, enhancing lending decisions.
- Improved customer retention through personalized financial products and services.
Healthcare Predictions and Outcomes
In the realm of healthcare, predictive analytics stands at the forefront of improving patient care. By analyzing patient data such as medical history, demographics, and even environmental factors, healthcare providers can anticipate health risks among populations. This approach enables proactive measures, resulting in better health outcomes.
For example, hospitals can anticipate patient influx during flu seasons and manage staffing accordingly, thereby enhancing operational efficiency. Predictive models can also drive the development of personalized treatment plans that adjust to the individual needs of patients.
Important uses in healthcare comprise:
- Predicting patient admission rates, optimizing resource allocation.
- Identifying at-risk patients for preventive care.
- Managing chronic diseases through tailored treatment strategies.
Therefore, predictive analytics serves as a foundational pillar across industries, providing insights that drive efficiency, enhance decision-making, and ultimately lead to improved outcomes.
Challenges and Considerations
When businesses embark on the journey of leveraging predictive analytics through SAP Analytics Cloud, there's more to consider than just the tech itself. There are certain challenges and considerations that can either propel an organization forward or hold it back. This section emphasizes why paying close attention to these factors is crucial for anyone looking to make data-driven decisions effectively. Understanding and addressing them helps mitigate risks, optimize outcomes, and ensures that insights derived from analytics are reliable and actionable.
Data Quality Issues
From the get-go, data quality emerges as a paramount concern. If the data feeding into predictive models is flawed, the predictions themselves can lead decision-makers astray. Think of it like trying to bake a cake with a handful of bad eggs; the entire endeavor can collapse.
To tackle data quality, organizations need to invest in rigorous data governance practices. This involves:
- Data Cleansing: Regularly checking and cleaning data sets to remove inaccuracies and inconsistencies.
- Standardization: Implementing uniform formats for data entries, making it easier to integrate and analyze.
- Validation: Continuously validating data sources to affirm their reliability and relevance, particularly before crucial business decisions are made.
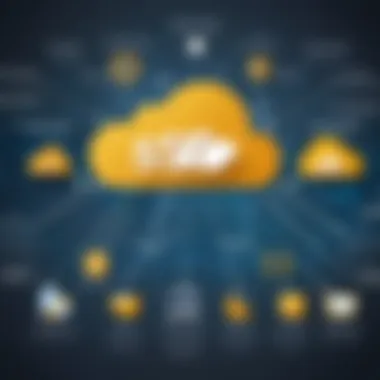
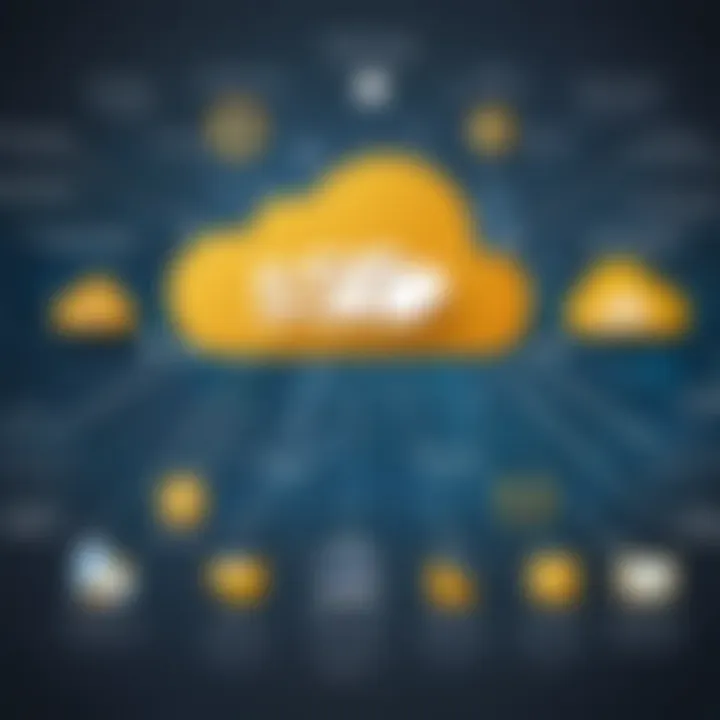
For companies utilizing SAP Analytics Cloud, leveraging built-in tools for data modeling can make this process smoother, but it requires a committed approach to maintaining high data integrity.
Algorithm Bias and Ethical Concerns
As algorithms analyze data patterns, one must also tread carefully around biases that might skew predictions. It’s essential to remember that algorithms are only as good as the data they learn from, and biased data will lead to biased outcomes. For instance, in a retail setting, if historical purchase data disproportionately reflects a particular demographic, then marketing strategies developed from that data may exclude other valuable customer segments inadvertently.
To address potential algorithm bias, organizations need to:
- Diverse Data Collection: Ensure that the data set encompasses a broad spectrum of demographics and behaviors, rather than focusing oonly on what is readily available.
- Regular Audits: Conduct audits of predictive models to assess fairness in outcomes, adjusting as necessary to prevent unfair advantages or disadvantages.
- Ethical Frameworks: Establish ethical guidelines that dictate how data is used and how outcomes are interpreted, ensuring respect for users and stakeholders.
In the realm of predictive analytics, addressing these challenges thoughtfully not only builds trust with the end-user but also fosters a culture of responsible data usage within an organization.
"Data-driven decisions are powerful, but they must be built on a foundation of quality data and ethical practices."
Best Practices for Implementing Predictive Analytics
When diving into the realm of predictive analytics, especially with the offerings from SAP Analytics Cloud, it’s paramount to establish a solid framework. Best practices serve as the bedrock upon which organizations can build effective predictive models. Understanding these principles can dramatically improve outcomes, ensuring that businesses not only keep up with the competition but lead it.
Setting Clear Objectives
Establishing clear objectives is akin to laying down the map before embarking on a journey. Without direction, you're just wandering about, hoping to stumble upon a solution. This clarity involves defining what you want to predict and why it holds significance for your organization. Key considerations include:
- Alignment with Business Goals: Your objectives should resonate with the overarching business strategy. Whether it’s improving customer satisfaction, optimizing costs, or boosting product delivery, those goals need to be crystal clear.
- Measurable Outcomes: It’s essential to frame your objectives in measurable terms. Use key performance indicators (KPIs) that allow you to evaluate success. For instance, instead of saying "increase sales," aim for "boost sales by 20% over the next quarter."
- Stakeholder Engagement: Involve relevant stakeholders in the objective-setting process. Their insights ensure that you consider various perspectives. This could mean collaborating with marketing, operations, or finance teams, to ensure holistic decision-making.
Getting this stage right can save a boatload of time and resources later on, as having specific goals can streamline the data selection process, model development, and result interpretation.
Continuous Learning and Improvement
The only constant in business and technology is change. Continuous learning and improvement are not just recommendations; they're essential to succeed in predictive analytics. As you implement your models, it’s important to embrace agility and adaptability. Here are some elements to consider:
- Iterative Analysis: After deploying your predictive models, don't just kick back and relax. Engage in regular reviews of model performance. This iterative analysis process allows you to refine algorithms and adapt to new data. If a model isn't performing as expected, diagnose the issue and adjust accordingly.
- Feedback Loops: Establish strong feedback channels from users and stakeholders. Understanding how well predictions work in real-world scenarios will help refine your approach. For example, if a sales prediction model consistently misses the mark, gather insights on why—there may be underlying factors you overlooked.
- Skill Development: Encourage continuous education for your team. Predictive analytics is a rapidly evolving field, so keeping up with fresh trends, tools, and methodologies guarantees that you’re always a step ahead. Workshops, webinars, and online courses can serve as valuable resources for upskilling.
"Adaptability is about the powerful difference between adapting to cope and adapting to win."
Implementing these best practices ensures that businesses utilizing SAP Analytics Cloud not only benefit from predictive analytics but also cultivate a culture of innovation and improvement. By setting the right objectives and fostering an environment of continuous learning, organizations can maximize their predictive capabilities and drive real business value.
Future Trends in Predictive Analytics
Navigating the landscape of predictive analytics brings to light various currents that are reshaping how organizations harness data. This section delves into future trends that will influence how businesses utilize SAP Analytics Cloud to tap into predictive capabilities. It's not just crucial—it’s imperative for companies looking to stay ahead of the curve in an increasingly data-centric world. The integration of advanced technologies and methodologies is paving the way for innovative approaches in predictive analytics, thereby unlocking new levels of insight and operational efficiency.
AI and Machine Learning Integration
As organizations shift towards more intelligent systems, the integration of AI and machine learning into predictive analytics is becoming a significant theme. The capability to breed algorithms with extensive datasets enables businesses to fine-tune their forecasting models.
- Enhanced Accuracy: Machine learning algorithms can adapt and learn from new data, resulting in improved accuracy over time. By constantly analyzing patterns and trends in real-time, organizations can expect dynamic adjustments in predictions.
- Automation: The reliance on manual processes for data analysis is dwindling. AI helps automate data cleansing and model creation, freeing up valuable time for analysts to focus on strategy rather than getting lost in data.
- Advanced Insights: With AI's ability to process vast amounts of data, companies can unearth correlations and insights that may not be immediately obvious to human analysts.
For businesses using SAP Analytics Cloud, harnessing AI not only streamlines operations but also creates a more robust predictive model that can respond to changes in the market instantly.
"The rise of AI and machine learning isn't just a trend; it's a transformation in how we think about data and its potential."
Real-Time Analytics Developments
The demand for real-time analytics is surging. Businesses are increasingly leaning on the ability to make decisions instantaneously based on the freshest data available. This shift is particularly relevant in fast-paced industries where every second counts, such as e-commerce and finance.
- Quick Decision-Making: Real-time analytics allows professionals to make immediate decisions, which can be the difference between seizing an opportunity or letting it slip through their fingers.
- Adaptive Strategies: With real-time insights, strategies can be adjusted on the fly. This adaptability allows companies to retain a competitive edge as they respond to market changes more swiftly than their competitors.
- Proactive Risk Management: Detecting anomalies or changes in data patterns as they happen aids in risk assessment. Organizations can act before small issues escalate into significant problems.
In the realm of SAP Analytics Cloud, real-time analytics capability complements predictive models by providing a richer context through dynamic data visualization, enabling stakeholders to draw actionable insights with clarity.
Ending
In this article, we've journeyed through the intricacies of leveraging predictive analytics with SAP Analytics Cloud. This conclusion serves to reinforce the major themes and insights that emerged, spotlighting the transformative potential predictive analytics has on modern businesses. By harnessing this technology, organizations find themselves not only able but equipped to face the unpredictable winds of change that characterize today’s market landscapes.
Recap of Key Insights
To recap what we learned:
- Strategic Decision-Making: Predictive analytics empowers businesses to make better decisions by providing them the tools to forecast outcomes based on historical data. Companies like Netflix utilize predictive insights to suggest what content users might enjoy based on their viewing habits.
- Enhanced Operational Efficiency: Automating data analytics reduces time spend crunching numbers and allows employees to focus on value-added activities. When companies analyze customer buying patterns, for instance, they can manage inventory effectively, thus cutting costs.
- Real-World Applications: From retail to healthcare, industries are employing predictive analytics to address specific challenges and improve service quality. In healthcare, hospitals might predict patient readmission rates, enabling them to tailor their post-discharge plans accordingly.
- Future Potential: As trends like AI and machine learning evolve, the integration of these technologies within SAP Analytics Cloud opens up further avenues for predictive analytics, suggesting that we haven't seen the full potential yet.
"Data doesn’t lie, but people can misunderstand it. Predictive analytics clears this fog."
These insights signify not just an understanding of what predictive analytics is but how businesses can deploy these principles to gain a competitive edge.
Encouragement to Embrace Predictive Analytics
As businesses tiptoe into an increasingly data-driven world, embracing predictive analytics should not just be considered—it's a must. Companies sitting on the fence about adopting such technology risk remaining static while their competitors fly ahead.
Moreover, early adoption is often related to a greater return on investment. There's no need to overthink it. The step forward can start small with exploratory use cases that progressively morph into larger implementations. Here are some encouragements to make the leap:
- Start With Clear Goals: Determine what you want to achieve with predictive analytics, whether it’s improving marketing effectiveness or optimizing overhead costs. A calculated approach produces better outcomes.
- Involve The Right Stakeholders: Engaging IT and business teams in tandem brings valuable perspectives. This collaboration ensures that projects align with business goals and technological capabilities.
- Invest In Training: Elevating the skill set of teams can enhance the effectiveness of analytics tools. The more your staff know, the more value they can extract.
- Continuous Experimentation: Don’t be afraid to pivot if a model isn’t working. Data has its quirks, and it is vital to continuously evaluate and adapt according to findings.
The advantages that predictive analytics afford are numerous, yet they manifest most significantly for organizations that actively commit to embracing this change. Using SAP Analytics Cloud as a foundational framework only enhances this journey, opening doors to possibilities previously thought unattainable.