Exploring the Best Free Text Analytics Tools Available
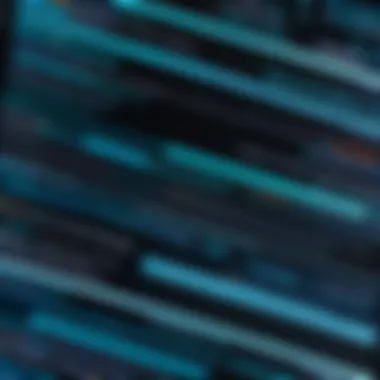
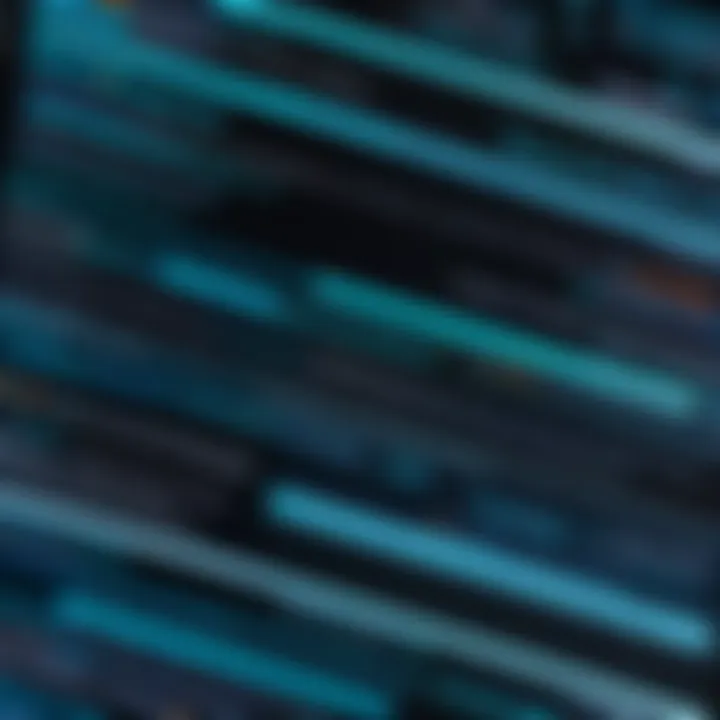
Intro
In the age of information overload, businesses, researchers, and professionals alike are seeking effective ways to derive meaning from vast amounts of text data. Free text analytics tools have emerged as essential software solutions that allow individuals to sift through mountains of unstructured information, extracting valuable insights while simplifying their decision-making processes.
These tools are particularly relevant today as organizations increasingly depend on big data to drive strategies, identify trends, and enhance customer interactions. Understanding how to leverage these resources can provide a significant edge in various sectors, from marketing to healthcare, by facilitating a deeper comprehension of consumer behaviors and market dynamics.
This article aims to serve as your compass in navigating the world of free text analytics tools. We'll explore numerous software options, examine their functionalities, compare features, and highlight their applications in real-world scenarios. By the end, you will be well-equipped to make informed choices regarding these powerful tools.
Understanding Text Analytics
Text analytics stands at the forefront of modern data analysis, bridging the gap between unstructured text data and actionable insights. In an era where information overload is a common challenge, understanding text analytics becomes crucial for organizations aiming to derive value from vast amounts of data. This section highlights key points about definition, importance, and essential concepts, which collectively inform the broader discussion of free text analytics tools.
Definition and Importance
At its core, text analytics refers to the computational methods used to convert text into meaningful data. It involves the extraction of pertinent information from unstructured sources, such as social media posts, customer feedback, and academic papers. The significance of text analytics lies in its ability to uncover trends, sentiments, and patterns that would otherwise remain hidden. For organizations, this means not merely accessing vast data repositories but also utilizing them effectively in decision-making processes, enhancing customer experiences, and identifying new market opportunities.
Key Concepts in Text Analytics
The field is rooted in several key concepts that underpin how text analytics tools operate and the value they provide. Let's explore some of the most foundational ideas:
Natural Language Processing
Natural Language Processing (NLP) is a branch of artificial intelligence dedicated to the interaction between computers and human language. Its role in text analytics is fundamental, as it empowers tools to understand and process human languages in a meaningful way. A distinguishing characteristic of NLP is its ability to analyze language contextually, which allows for more accurate interpretations of text.
One unique feature of NLP is its capacity to handle synonyms and polysemyâwords that have multiple meaningsâmaking it a beneficial choice in any text analytics endeavor. It fosters a nuanced understanding of sentiment and intent, providing deeper insights into user data. However, challenges persist, including issues with sarcasm or cultural idioms, often leading to misinterpretations in analysis, which can complicate outcomes in practical applications.
Sentiment Analysis
Sentiment analysis dives deeper into determining the emotional tone behind a series of words, often gauging whether the sentiment expressed in a piece of text is positive, negative, or neutral. This capability is crucial for businesses looking to understand customer opinions about their products or services, giving them a competitive edge by aligning offerings with consumer sentiment. A key feature of sentiment analysis tools is their scalability, allowing for the analysis of massive datasets across different platforms in real-time.
However, while sentiment analysis is incredibly powerful, it does come with its pitfalls. The subtleties of human emotions can lead to misclassification when tools interpret sarcastic remarks or complex sentiments. Such limitations highlight the need for continuous advancements in algorithms to improve accuracy in competitive contexts.
Entity Recognition
Entity recognition is a specific function of text analytics that involves identifying and categorizing key elements within textâlike names, organizations, locations, and expressions of time. It plays a vital role in knowledge extraction, drawing attention to essential elements in large data sets that could inform strategic decisions.
A notable feature of entity recognition systems is their adaptabilityâthey can be customized to recognize domain-specific entities, which is particularly useful in fields such as healthcare or finance. However, this brings challenges as well, as training these systems requires substantial high-quality annotated data for effective learning, and the sophistication of language in these domains can complicate matters.
"Understanding the intricacies of text analytics is not just an academic pursuit; itâs a business necessity in todayâs data-driven landscape."
In sum, comprehending text analytics involves more than just knowing about the tools; it requires looking into the theories and principles that shape their usage. By grounding the discussion in defined terms, the importance of this field becomes remarkably clearâparticularly as businesses seek to thrive in environments rife with continuous data generation. As we venture into the evolution of text analytics tools, these foundational concepts will serve as a framework for appreciating the innovations and improvements made in this ever-evolving discipline.
Evolution of Text Analytics Tools
The journey of text analytics tools is akin to a winding road that has adapted and transformed over time, adjusting to the demands of various industries. Understanding the evolution of these tools offers insights not only into past methodologies but also into the innovations shaping the present and future of text analytics. Here, we explore two critical aspects: a historical overview of how these tools came to be and the technological advancements that have propelled their growth.
Historical Overview
Text analytics, in its infancy, relied heavily on basic statistical techniques. The early days could be likened to learning to walk; foundational methods such as frequency counts and simple keyword searches emerged to help sift through large volumes of text. These rudimentary approaches laid the groundwork for more refined techniques.
A notable milestone occurred in the early 2000s, when Natural Language Processing (NLP) began to gain traction. The development of algorithms that could parse human language kicked text analytics into a higher gear. This evolution mirrored changes in society as communication became increasingly digital, with the explosion of content on the internet.
"As the digital universe continues to expand, so too does the need for robust text analytics tools that can make sense of the noise."
By the mid-2010s, sentiment analysis became a buzzword. Companies were no longer just measuring how many times a word appeared; they began analyzing the sentiment behind words in social media posts, reviews, and other digital communications. This period saw the entry of many startups into the text analytics domain as businesses recognized the strategic value of understanding consumer sentiment.
Technological Advancements
As the saying goes, "Out with the old and in with the new." The technological innovations within text analytics tools showcase just that. The rise of machine learning and artificial intelligence marked a seismic shift in how text data was analyzed. Unlike traditional methods that required manual input, modern tools can learn patterns from massive datasets, enabling them to predict outcomes based on real-world scenarios.
Recent advancements also include enhancements in machine learning frameworks, which have made powerful analytics more accessible. Open-source libraries, such as TensorFlow and Natural Language Toolkit, illustrate this trend effectively. They facilitate a level of experimentation that was previously limited to large corporations with hefty budgets.
Moreover, cloud computing has made it much easier for businesses to store and process large amounts of text data without investing in physical infrastructure. Companies can now scale their analytics efforts as needed, making powerful tools more financially viable for smaller businesses.
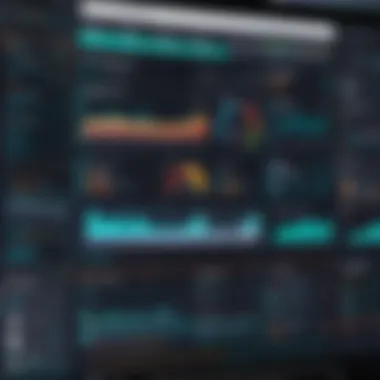
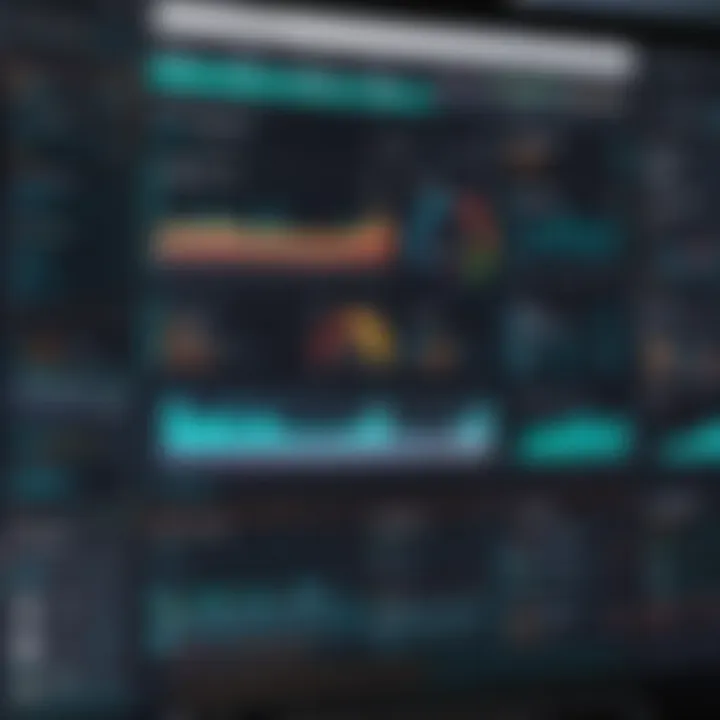
In short, the evolution of text analytics tools has been marked by a gradual, yet pivotal shift from basic text manipulation methods to sophisticated, AI-driven analytical frameworks. It's a transformation that not only reflects advancements in technology but also highlights the growing importance of data in decision-making processes across various industries.
Criteria for Selecting Text Analytics Tools
When it comes to choosing the right text analytics tools, it's not just a simple pick from a hat. Thereâs a science to it, and understanding the criteria for selection can make all the difference in harnessing the power hidden within your data. Many tools promise the moon and the stars but fail to deliver when it comes to practical applications. Knowing what to look for will streamline your decision-making process and enhance the utility of the tools you choose.
Functionality and Features
First and foremost, functionality is king in the realm of text analytics. A toolâs core capabilities dictate how effectively it will serve your purpose.
- Key Functionalities: Look for tools that offer a rich blend of features such as text classification, sentiment analysis, and topic modeling. Each can address different needs, from analyzing customer sentiment to identifying trends in social media conversations. For example, a tool that can not only parse text but also generate actionable insights sets itself apart.
- User-friendly Features: Additionally, features like real-time processing and customizable dashboards elevate the user experience. Tools capable of handling varied data sources, such as social media posts, emails, or even chat logs, are also advantageous. The inclusion of visualizations to present data findings succinctly makes analysis less of a chore and more of a journey.
Ease of Use
Selecting a tool that wonât require you to climb Mount Everest to understand it is crucial. The phrase "user-friendly" might sound clichĂŠd, but in this context, it means everything.
- Intuitive Interfaces: A streamlined interface can save hours and prevent headaches. Look for tools that enable users to effortlessly navigate through features without the need for extensive training. Itâs worthwhile to experiment with demo versions or free trials to gauge this aspect firsthand.
- Documentation and Tutorials: Moreover, good documentation and readily available tutorials can be invaluable for easing users into the software landscape. A tool that provides these resources demonstrates commitment to user success.
Integration Capabilities
Imagine youâve found a textual analysis tool that performs wonders, but it cannot jive with your existing systemsâthat feels like finding a shiny penny in a muddy puddle.
- Compatibility: Tools need to fit into your current technological ecosystem smoothly. This includes compatibility with databases, workflows, and other software. An analytics tool should not stand alone; it must complement and enhance your existing systems.
- APIs and Plug-ins: Additionally, consider the presence of APIs or plug-ins that support automation and data transfers. This capability can significantly streamline your processes and enhance efficiency.
Support and Community
Finally, letâs address a factor thatâs often overlooked: support and community engagement with the tool. Choosing a tool that leaves you high and dry when problems arise is a recipe for frustration.
- Support Availability: Evaluate whether the tool has responsive customer support. Timely assistance can salvage critical analytical moments. An active support team that responds swiftly to queries can make all the difference in your day-to-day activities.
- Community Engagement: Look for vibrant communities on platforms like Reddit or dedicated forums where users share insights, tips, and support. A tool that fosters a strong community is likely to have a loyal user base, which can be advantageous for new users seeking guidance.
In summary, taking the time to evaluate these criteria isn't merely about ticking boxesâit's a strategic move that impacts how well you can leverage text analytics tools for your respective needs.
Overview of Popular Free Text Analytics Tools
In today's data-driven world, text analytics has seeped into various sectors, providing valuable insights and informing strategic decisions. Free text analytics tools have emerged as essential instruments for businesses, researchers, and analysts seeking to leverage unstructured data effectively. These tools allow users to distill vast amounts of information into actionable insights, making the exploration of popular options a critical part of understanding the landscape.
When considering free text analytics tools, it is vital to look at several key facets: functionality, accessibility, and user community support. Each tool comes with unique features tailored to different needs, so familiarizing oneself with their offerings is paramount. This landscape offers a variety of choices catering to individuals, teams, and organizations looking for robust solutions without breaking the bank.
Here, we delve into three notable tools, examining their functionalities and the specific use cases that highlight their contributions to text analytics. By understanding these instruments, one can make informed decisions aligned with specific needs and objectives.
Tool One: Overview and Features
Key Functionalities
This tool stands out for its ability to efficiently process and analyze large text datasets. Its core functionality includes natural language processing capabilities, which enable users to derive meaning and insights from text inputs. Additionally, it's equipped with advanced algorithms supporting entity recognition and sentiment analysis, which are crucial for businesses aiming to understand customer sentiments and brand perceptions.
A notable aspect of this tool is its user-friendly interface, which accommodates both beginners and experienced analysts. The customizable dashboards allow users to visualize data in various formatsâbe it graphs, charts, or word cloudsâfacilitating a clearer understanding of trends.
However, while the tool excels in many areas, it does have limitations regarding the depth of sentiment analysis, which may not be as fine-tuned as some paid options.
Use Cases
This tool has been widely adopted in areas such as marketing and customer support. For example, a marketing team might use it to gauge customer reactions to a new product launch by analyzing social media posts and reviews. The immediate feedback provides actionable insights that can be used to adjust marketing strategies promptly.
Moreover, this tool excels in monitoring brand mentions across various platforms, helping businesses to stay abreast of public sentiment. The strength lies in its real-time tracking, enabling responsive actions that can mitigate potential PR issues early.
Tool Two: Overview and Features
Key Functionalities
Another popular free text analytics tool is recognized for its robust data mining capabilities. It allows users to extract insights from extensive datasets swiftly, significantly enhancing productivity. The pattern recognition feature sets it apart, enabling businesses to discover trends over time that might otherwise remain hidden.
The tool also integrates Machine Learning algorithms, which can refine results as more data is added, thereby improving accuracy. This feature is particularly beneficial for businesses wishing to adapt their strategies based on emerging patterns.
However, integrating this tool into existing systems can pose challenges, especially for users unfamiliar with data science concepts. This could dilute its accessibility for some non-technical users.
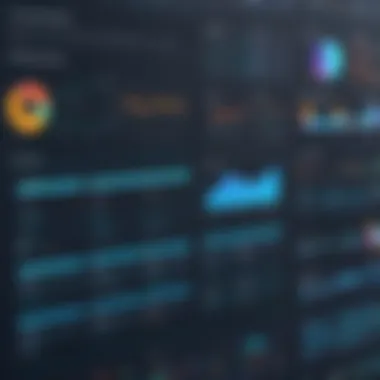
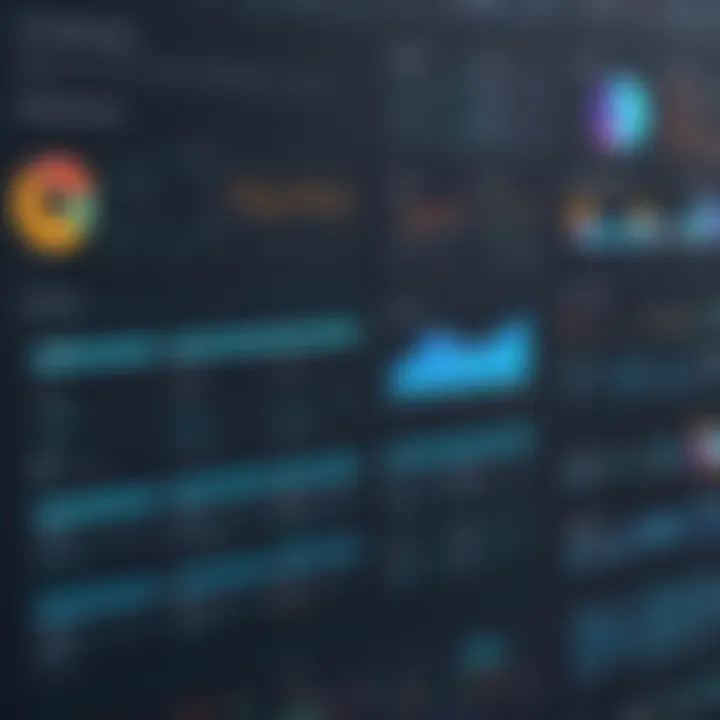
Use Cases
Organizations use this tool effectively in research environments, particularly in understanding consumer behavior through survey feedback analysis. A retail chain, for instance, can analyze customer feedback across multiple channels to determine which products require improvements.
Its versatility also makes it suitable for academic research, where scholars can sift through extensive literature to identify key recurring themes in their field of study. Nevertheless, users should be aware that due to its complexity, there might be a steeper learning curve associated with mastering all functionalities.
Tool Three: Overview and Features
Key Functionalities
This third contender is particularly noted for its emphasis on collaborative features, allowing multiple teams to work on text analytics projects simultaneously. It offers a shared workspace where users can collectively analyze data, share insights, and brainstorm solutions, which is crucial in a fast-paced business environment.
Another significant feature is the integration with external data sources, making it easy to pull in relevant information from an array of channels such as social media, emails, or internal documents. The toolâs API functionality allows for seamless integration with other platforms, making it a flexible choice for tech-savvy users.
One downside is its dependence on internet connectivity, which may not suit all users, particularly those in regions with unstable internet access.
Use Cases
Many marketing agencies leverage this tool to run comprehensive social media campaigns, where collaboration among team members is essential for brainstorming and executing strategies effectively. Its ability to integrate data from various sources means that user insights are not siloed but operate in a connected ecosystem.
Additionally, businesses using it for internal communication analysis can improve team productivity by identifying common pain points within the organization. However, for users outside of digital marketing who may not require continuous collaboration, this tool might not be as advantageous.
Overall, exploring the variety of free text analytics tools available not only broadens one's understanding of text analytics itself but also empowers users to make well-informed decisions about which tools best serve their specific needs.
Applications of Text Analytics Tools
Understanding the applications of text analytics tools is crucial for any organization looking to enhance its decision-making processes. Text analytics operates as a method of deriving insights from unstructured data. By sifting through large amounts of text â be it customer feedback, social media interactions, or news articles â businesses can identify trends, sentiments, and key themes that help inform strategies. In todayâs data-driven world, neglecting the potential of these tools could mean missing out on significant advantages and insights.
Business Intelligence
In the realm of business intelligence, text analytics tools play a pivotal role in shaping strategic decisions. By leveraging text data, companies can analyze customer reviews, employee feedback, or market reports to get a clearer picture of their operational strengths and weaknesses. For instance, when retailers analyze comments and reactions on review platforms, they can pinpoint which products generate the most buzz and which ones might need improvement.
- Sentiment Analysis: This is a core function where tools evaluate customer opinions and emotions expressed through written content. It enables businesses to gauge public perception accurately and pivot their marketing strategies accordingly.
- Trend Identification: Text analytics can surface emerging trends as they occur, allowing businesses to stay one step ahead of competitors. If a sudden increase in the demand for a new product is noted, quick responsive action can be taken.
Companies like Tableau have incorporated text analytics features into their platforms, allowing users to visualize and interpret large datasets that include text.
Customer Relationship Management
Text analytics enhances Customer Relationship Management (CRM) by providing valuable insights into customer interactions and behaviors. Companies can analyze communications, complaints, and compliments to create a more detailed customer profile. This evolving understanding helps personalize customer experiences and product offerings.
- Feedback Loops: By analyzing customer feedback, organizations can develop strategies that address common pain points. If a significant number of customers voice dissatisfaction about delivery times, it can trigger logistical adjustments.
- Predictive Modeling: The data harvested can also be used for predictive analytics. For example, if previous interactions indicate a customer's likelihood to purchase a new product based on sentiment, companies can reach out proactively with targeted promotions.
Using platforms like HubSpot allows businesses to automate responses and insights from text analytics, fostering improved customer interactions.
Market Research and Trend Analysis
The use of text analytics in market research provides an avenue for companies to make data-backed decisions. By integrating text data analytics into their research methodologies, businesses can access a wealth of information from various unstructured sources, such as social media posts, online reviews, and industry news.
- Competitor Analysis: Businesses can analyze competitor communication and identify gaps in the market. Understanding how competitors are positioned in the eyes of the public can offer critical advantages.
- Consumer Trends: Insights can be gathered that reflect changing consumer preferences or interests. If analysis shows a growing preference for vegan products in customer feedback, businesses can be quick to innovate or adapt their offerings.
In summary, organizations must leverage these tools actively to harness the strengths of text analytics, gaining valuable insights that directly influence their strategies and market positioning. The integration of text analytics helps not just in understanding data but in actively utilizing it for growth and innovation.
Challenges in Text Analytics
When discussing text analytics, it is vital to tackle the challenges that come with it. These hurdles are not just minor inconveniences; they can significantly impact the accuracy and efficiency of data analysis. As organizations increasingly rely on text analytics to gather insights, understanding these challenges allows for better strategic decisions. Recognizing these roadblocks provides a clearer path for selecting and deploying the right tools, ensuring that businesses can truly harness the power of unstructured data.
Data Quality Issues
Data quality is at the heart of text analytics. Poor data quality leads to unreliable insights; bad data is like a leaky bucketâit fails to hold the insights you're pouring in. This can stem from a variety of sources: for instance, inconsistent formatting, irrelevant information, and outdated content. If a tool processes text dodging these data pitfalls, the results may be skewed.
A few specific elements can exacerbate data quality issues:
- Inconsistent Terminology: Different teams using varying terms for similar concepts can lead to discrepancies. One might say "customer service reps," while another refers to "support agents."
- Incomplete Data: When datasets are missing crucial elements, the analytics may present an incomplete picture. It's like reading a book with missing pages; key plot points are just not there.
- Noise and Redundancy: Unwanted information, or 'noise,' can drown out valuable insights. For example, advertisements or irrelevant social media posts can clutter data sets.
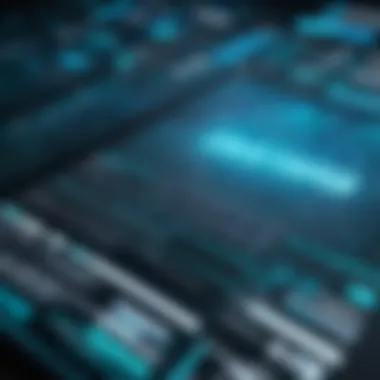
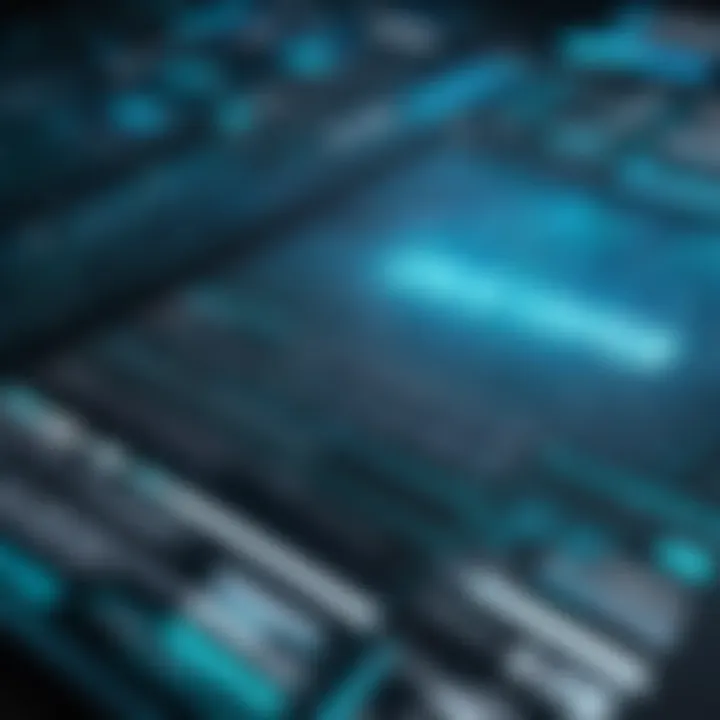
Organizations must invest time to clean and curate the data before analyzing, providing a stronger foundation for analytics tools to operate effectively.
Language and Dialect Variations
In an increasingly globalized world, language and dialect variations pose distinct challenges. Text analytics tools may struggle with nuances, idioms, or even slang specific to regions. For instance, whatâs considered "cool" in one culture might not be understood elsewhere.
Some of the key issues include:
- Regional Dialects: Different geographical areas may have their own slang terms. A word can mean quite different things depending on the context.
- Language Barriers: Tools might not support multiple languages equally, leading to biased data analysis. For example, data from English-speaking regions may hold more value than data from other languages.
- Polysemy and Homonymy: Words that have multiple meanings can lead to confusion during text processing. Take the word "bat"âis it a flying mammal or sports equipment? The context is everything here.
Addressing these language-related challenges often requires customizing the tools or incorporating advanced Natural Language Processing techniques.
Scalability Concerns
Scalability in text analytics refers to the ability of a tool to handle increasing amounts of data efficiently. As organizations grow, their data needs expand too. This can create bottlenecks if the chosen text analytics tool doesnât scale alongside the growth.
Here are several factors to consider:
- Volume of Text Data: As businesses generate more text data from emails, documents, and social media, tools must be able to analyze larger datasets without crashing. Otherwise, the system becomes as useful as a paper plane in a thunderstorm.
- Processing Speed: In a fast-paced environment, the time taken to analyze data is crucial. A tool that processes data slowly may lead to missed opportunities.
- Integration with Other Systems: Ensuring that the analytics tool can fit seamlessly into existing data systems is necessary for scalability. A tool that is incompatible with current software could be a recipe for disaster.
Thus, when selecting text analytics tools, organizations need to consider not just their current data handling needs but their future expansion goals.
Understanding the challenges of text analytics is essential for leveraging its potential. By being aware of data quality issues, language and dialect variations, and scalability concerns, businesses can make informed decisions and ensure successful outcomes.
Future Trends in Text Analytics
As the landscape of text analytics continues to evolve, understanding the future trends in this field becomes paramount. This section highlights how innovations and advanced methodologies will shape the use and functionality of text analytics tools. Identifying these trends not only informs tech-savvy individuals and business professionals about what to expect but also aids in preparing for the implications that might arise in various settings.
AI and Machine Learning Integration
The integration of artificial intelligence and machine learning into text analytics is more than just a buzzword; it's a game changer. With advances in natural language processing, systems can now comprehend text with greater accuracy than ever before. For instance, ML algorithms can detect subtle sentiment nuances in customer feedback that traditional tools might overlook.
- Predictive Analysis: Machine learning is increasingly being used for predictive analysis, where algorithms analyze historical data to forecast future trends. This can be particularly useful for businesses aiming to preempt customer needs by analyzing sentiments in social media.
- Contextual Understanding: AI has the capability to understand context better, making it easier to analyze idiomatic expressions or specialized industry terms that would confuse a simpler text analytics tool.
"As text analytics tools evolve, the integration of AI and machine learning will enable a more nuanced understanding of human language, ultimately driving better business decisions."
The adaptability of these systems allows them to learn from new data continually, ensuring that organizations stay ahead of the curve.
Real-Time Analytics
Real-time analytics has transformed how organizations interact with data. In today's fast-paced environment, the ability to assess and act on data in real-time can provide a significant competitive edge. Text analytics tools that incorporate real-time capabilities are invaluable for various applications:
- Social Media Monitoring: Businesses can achieve immediate insights into customer sentiment and brand perception. This is crucial for tailoring marketing strategies or addressing any potential PR crises quickly.
- Customer Support: Tools that analyze customer interactions as they happen allow businesses to adapt their responses in real-time. This leads to improved customer satisfaction and quicker resolution rates.
Real-time analytics not only sharpens decision-making processes but also supports more agile business models, allowing companies to pivot swiftly when necessary.
Enhanced User Experiences
In looking to the future, enhancing user experiences with text analytics tools ranks high on the list of priorities. Users increasingly demand intuitive interfaces and meaningful visualizations that help them make sense of complex data. Key aspects include:
- User-Centric Design: As understanding grows, so does the expectation for tools that are not just powerful but also easy to navigate. A focus on user experience involves designing interfaces that prioritize ease of use, helping professionals extract insights with minimal effort.
- Customization Options: More tools are offering customizable dashboard features, allowing users to choose how they want to visualize their data. This leads to more effective insights while catering to individual preferences.
- Interactive Features: Integrating features that allow users to manipulate data directly can lead to dynamic insights. For example, being able to filter datasets on-the-fly during a presentation can elevate discussions significantly.
As users demand more from text analytics tools, the focus will shift to enhancing the interactions they have with these tools. The goal is to foster an environment where insights are easily obtainable and actionable, supporting informed decision-making.
Finale
In wrapping up our discussion on free text analytics tools, itâs clear that these solutions are not just supplementary software; they have become vital assets across various sectors. The significance of these tools lies in their capacity to transform unstructured text data into coherent insights, which can lead to more informed decision-making. As organizations increasingly rely on data-driven strategies, understanding the implications and applications of text analytics is essential.
Recap of Key Points
- Functionality and Features: We discussed how different tools provide various functionalities such as sentiment analysis, entity recognition, and visualization features.
- Ease of Use: User-friendly interfaces are crucial for adoption. If a tool is too complex, organizations might overlook its potential.
- Integration Capabilities: The ability to combine these tools with existing systems can significantly enhance workflow efficiency.
- Support and Community: A strong user community and robust support system are key indicators of a toolâs reliability and long-term viability.
- Business Applications: From market research to customer relationship management, the data processed can be a game-changer for strategic planning and execution.
- Challenges: Awareness of challenges, like data quality issues and scalability, is important for a realistic approach towards implementation.
By reflecting on these crucial points, readers can appreciate the breadth of functionalities offered by free text analytics tools and how they can be optimally leveraged to meet unique business needs.
Final Thoughts on Free Text Analytics Tools
The landscape of text analytics is perpetually evolving. The integration of advanced technologies like AI and machine learning is setting the stage for more sophisticated analytics solutions. As businesses continue to collect vast amounts of data, having the right text analytics tools can spell the difference between stagnation and growth.
"In the era of information overload, the ability to derive insight from data is not just an advantage; it's a necessity."
Looking ahead, organizations must not only invest in these tools but also foster a culture of continuous learning to keep up with emerging trends. The tools available today are powerful, but they must be aligned with strategic objectives for meaningful impact. This will not only ensure effective utilization but also position businesses to harness the full potential of their textual data effectively.