In-Depth Exploration of Data Analytics Programs
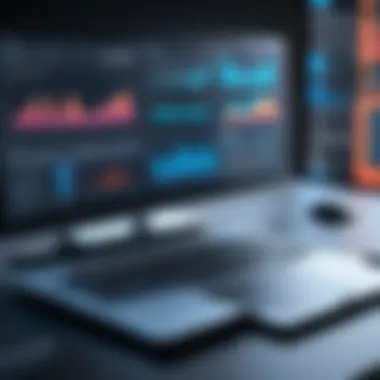
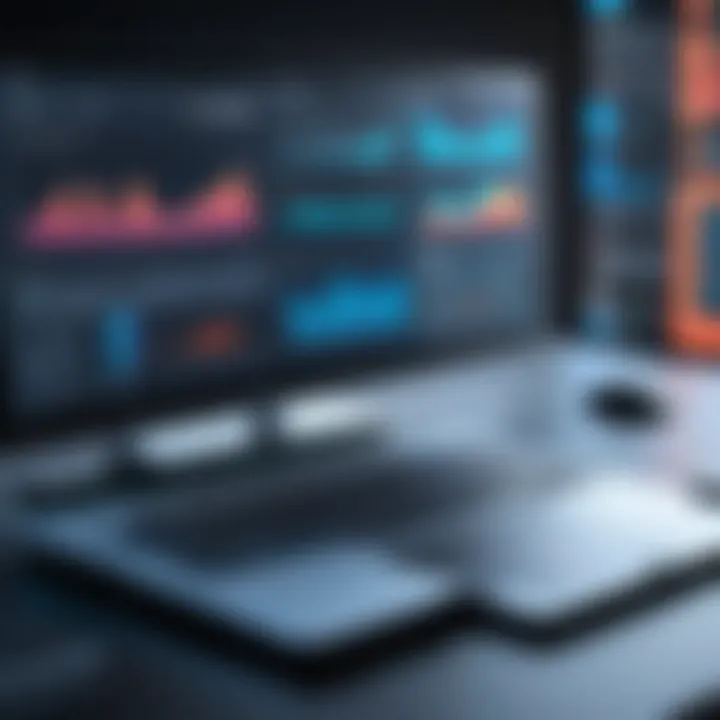
Intro
In the current digital era, the significance of data analytics cannot be overstated. Organizations of all sizes are harnessing data as a key asset, driving strategies and operational decisions. Data analytics software plays a central role in this landscape, providing the tools necessary to transform raw data into valuable insights. This article aims to explore various data analytics computer programs, discussing their features, utility, and importance in shaping analytical capabilities.
Software Overview and Benefits
Various data analytics software solutions exist in the market today, each offering unique features tailored to meet specific business needs. Programs like Tableau, Microsoft Power BI, and SAS Analytics are among the major players, each boasting distinctive functionalities that cater to different analytical requirements.
Tableau excels in visualizing data through its user-friendly interface, enabling users to create interactive dashboards easily. Microsoft Power BI integrates seamlessly with the Office suite, making it popular for organizations that are already embedded in the Microsoft ecosystem. SAS Analytics is widely recognized for its statistical analysis capabilities, particularly useful in sectors requiring rigorous data validation and modeling.
The benefits of these software solutions extend beyond just data visualization. By using these tools, businesses can:
- Make data-driven decisions, enhancing accuracy in forecasts.
- Identify trends and patterns in large data sets, allowing for timely interventions.
- Improve operational efficiency through streamlined reporting processes.
These advantages are crucial for driving business growth and fostering a data-centric culture.
Pricing and Plans
The pricing structures for data analytics software vary significantly based on features, capabilities, and target audience. For example, Tableau offers a subscription model with prices starting at $70 per user per month for their Professional edition. Power BI, on the other hand, provides a more budget-friendly option at $9.99 per user per month, appealing to small businesses and individual users.
When comparing these prices, itβs important to consider not just the initial cost but also the total value offered by each program. While SAS Analytics may charge a premium due to its extensive functionalities, organizations that require complex data manipulation might find the investment worthwhile.
Performance and User Experience
Assessing the performance of data analytics software is essential for understanding its practical applications. Speed, reliability, and user interface are all critical factors. Tableau is often praised for its fast rendering capabilities, allowing users to visualize large datasets without noticeable delays. Power BI's performance also shines, particularly for organizations familiar with the Microsoft ecosystem.
User feedback is essential in evaluating usability. Most users appreciate Tableau's drag-and-drop interface, which simplifies the process of creating visualizations. In contrast, some might find SAS Analytics to have a steeper learning curve, given its advanced statistical capabilities. Still, such complexity can be beneficial for power users accustomed to working with intricate data models.
Integrations and Compatibility
Integrations with other tools and platforms can greatly enhance the utility of data analytics software. Tableau, for instance, supports integration with various databases like SQL Server and Salesforce, allowing companies to use existing data investments effectively. Microsoft Power BI offers strong integrations with all Microsoft applications, making it a natural choice for businesses already using those tools.
Assessing compatibility is also important. Many software solutions are cloud-based, which allows for greater accessibility across operating systems. Tableau and Power BI operate efficiently on Windows, while also providing options for access on Mac systems.
Support and Resources
Customer support options vary across data analytics software. Tableau provides extensive support resources, including community forums and training sessions. Their FAQ and documentation are also comprehensive, helping users troubleshoot issues efficiently.
Additional resources such as video tutorials, webinars, and user guides can further enhance the user experience. Microsoft Power BI's online community is particularly active, offering forums where users can ask questions and share insights.
"The right data analytics tools can transform the way an organization functions, leading to improved decision-making and operational efficiencies."
Intro to Data Analytics
Data analytics is a crucial component for organizations striving for efficiency and growth in an increasingly data-driven world. Understanding its principles and applications can empower decision-makers to leverage data effectively. This article aims to provide a thorough exploration of data analytics computer programs, emphasizing their importance and how they drive insightful outcomes.
Definition and Importance of Data Analytics
Data analytics refers to the systematic computational analysis of data. It involves inspecting, cleansing, transforming, and modeling data with the goal of discovering useful information, informing conclusions, and supporting decision-making. Its importance cannot be overstated; as businesses collect vast amounts of data, the ability to analyze this data increases their competitive edge. Data analytics helps identify trends, optimize operations, and improve customer experiences. Additionally, businesses can make data-driven decisions, reducing guesswork and enhancing overall strategic planning.
Role of Data Analytics in Decision Making
Data analytics plays a vital role in modern decision-making processes. By utilizing sophisticated algorithms and computational techniques, organizations can interpret complex data sets. This leads to clearer insights that influence choices at various levels, from day-to-day operations to long-term strategies. The integration of data analytics into the operational framework enables organizations to:
- Predict Outcomes: Analyzing past behavior helps forecast future trends.
- Identify Opportunities: By uncovering hidden patterns, companies can find new markets or enhance existing products.
- Mitigate Risks: Understanding data trends can help anticipate potential challenges, allowing for proactive measures to be taken.
Understanding Data Analytics Computer Programs
Data analytics computer programs are essential tools in today's data-driven environment. Understanding these programs enables businesses to leverage data for better decision-making, operational efficiency, and strategic growth. The proliferation of data across industries necessitates effective ways to analyze and interpret this information.
Data analytics software helps organizations extract valuable insights from raw data. It simplifies complex analysis, allowing users to focus on data interpretation rather than technical processing. Moreover, by enhancing data accessibility, these programs foster a culture of data-driven decision-making at all levels of an organization.
Overview of Data Analytics Software
Data analytics software encompasses a wide range of applications designed to process, analyze, and visualize data. These programs can cater to various needs, from simple data sorting to complex statistical analysis.
Some of the key functionalities of data analytics software include:
- Data Integration: Combining data from multiple sources into a cohesive format.
- Data Mining: Discovering patterns and relationships in large datasets.
- Statistical Analysis: Applying mathematical techniques to interpret data effectively.
- Visualization: Transforming data into visual formats such as charts or dashboards to make insights easily comprehensible.
Understanding how these software applications function allows businesses to select solutions that align with their specific needs and goals.
Categories of Data Analytics Programs
Data analytics programs can be broadly classified into several categories, each serving distinct purposes:
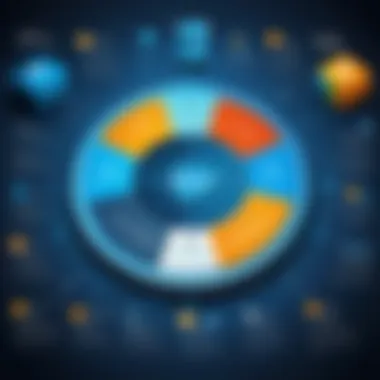
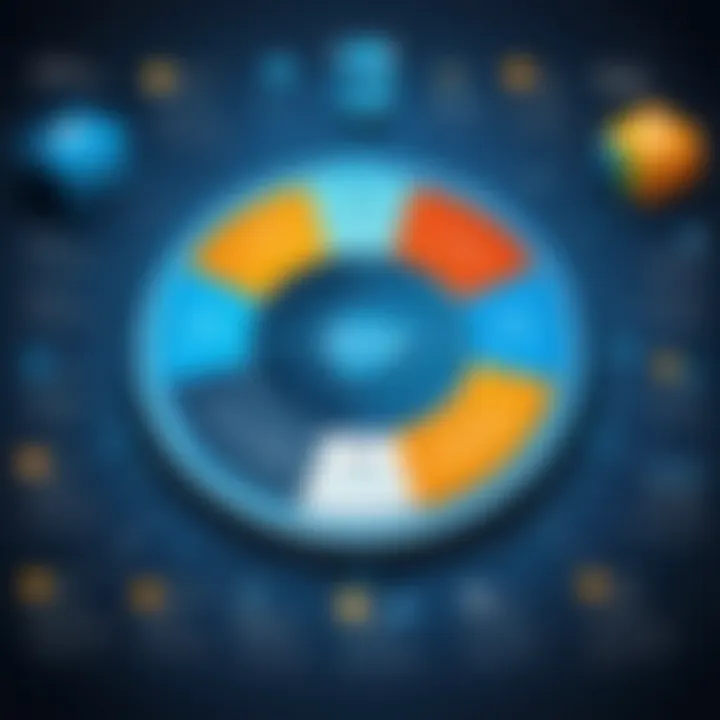
- Commercial Software Solutions: These include high-end products like Microsoft Power BI and SAS. They offer robust functionalities but often come with a significant cost.
- Open Source Tools: Programs like R and Python libraries are popular among data scientists. They provide flexibility and are often free, which is attractive for small businesses or individual users.
- Cloud-Based Services: Applications like Tableau Online allow users to access analytics from anywhere. This is especially useful for teams spread across different locations.
- Business Intelligence Tools: Software such as Tableau and QlikView focus on analyzing business data. They often include features for reporting and dashboards, which help stakeholders visualize key metrics in real-time.
Understanding these categories helps organizations make informed decisions based on their specific analytical needs, budget, and desired outcomes.
Fundamental Features of Data Analytics Programs
The functional aspects of data analytics programs are crucial for effectively leveraging data in decision-making processes. Understanding these fundamental features can spell the difference between a successful analytical endeavor and a fruitless one. In a world awash with information, having the right tools to handle data can improve productivity, enhance accuracy, and ultimately guide strategic decisions. The role of these features is not merely operational; they are pivotal in shaping insights that drive organizational growth.
Data Collection and Integration
Data collection is the first step in analytics. It involves gathering data from various sources including databases, cloud services, and real-time feeds. Effective data collection ensures that analysts have the necessary information to work with. Integration relates to the ability to combine this data into a single, coherent dataset. When datasets come from multiple sources, integration can pose challenges.
A successful integration process helps in unifying data formats. It prevents issues stemming from inconsistencies. For instance, organizations may use a customer relationship management tool like Salesforce alongside a data warehouse such as Amazon Redshift. Ensuring these systems communicate well is vital.
"Data collection and integration enable robust analytics, ensuring no vital information is overlooked."
- Benefits of Effective Data Collection and Integration:
- Promotes consistency in analysis
- Enables comprehensive insights by avoiding data silos
- Facilitates tracking of data changes over time
Data Cleaning and Preparation
Once data is collected, it needs to be prepared for analysis. This phase is often considered the most time-consuming. Data cleaning involves identifying and correcting errors in the dataset. Common issues include duplicates, missing values, and incorrect formatting. Inaccurate data can lead to flawed conclusions, making this step essential.
Preparation goes beyond cleaning. It involves transforming data into a suitable format for analysis. Analysts may need to normalize data or create new variables. Creating a streamlined dataset is crucial because it allows algorithms to run effectively without encountering roadblocks.
- Challenges in Cleaning and Preparing Data:
- Identifying the correct cleaning techniques
- Balancing thoroughness with efficiency
Data Visualization Techniques
Data visualization is the final feature that significantly enhances analytics. It translates complex data into visual formats such as charts, graphs, and dashboards. The primary goal is to identify patterns and trends that might be difficult to discern in raw data. Good visualization permits stakeholders to grasp insights quickly.
Different tools, like Tableau or Microsoft Power BI, provide varied visualization capabilities. An effective visualization can foster understanding among diverse usersβbe it a data scientist or a business executive. Choosing the right visualization type is often critical; what works for one dataset might not be effective for another.
- Key Considerations for Data Visualization:
- Clarity and simplicity in presenting information
- Ensuring accuracy to avoid misleading representations
Understanding these fundamental features equips users with the knowledge to make informed choices when working with data analytics programs. It underscores the importance of not just having data, but having the right systems and methodologies in place to derive meaningful insights.
Popular Data Analytics Computer Programs
Data analytics computer programs form the backbone of effective data-driven strategies. Their role is not just about manipulating data; it is about transforming that data into actionable insights. By utilizing various software solutions, organizations can significantly improve decision-making processes and gain a competitive edge in their respective fields. This section highlights the importance of popular data analytics programs, focusing on both commercial solutions and open-source tools.
Commercial Software Solutions
Commercial data analytics software solutions are essential for businesses that require robust tools with substantial support and resources. Tools like Tableau, Microsoft Power BI, and SAS are leading examples in this category. These programs are designed to cater to the needs of various industries, offering comprehensive functionalities that assist with business intelligence, predictive analytics, and reporting.
The advantages of commercial software include:
- Robust Customer Support: Professional support is often available, ensuring that organizations can resolve issues quickly.
- User-Friendly Interfaces: Many commercial products prioritize ease of use, aiding in user adoption.
- Regular Updates: Vendors typically provide ongoing software updates, enhancing functionality and security.
- Advanced Features: These programs often come with powerful features, including artificial intelligence capabilities and advanced analytics that are not always present in open-source tools.
Companies must consider the licensing costs associated with these solutions, which can vary significantly. Organizations should assess their needs carefully to select a software solution that aligns with their objectives without incurring unnecessary expenses.
Open Source Tools
Open-source data analytics tools offer another pathway for organizations looking to harness the power of data without incurring significant costs. Examples like R, Python, and Apache Spark serve as powerful alternatives to commercial products. These programs are often community-driven, with countless plugins and libraries developed by users worldwide.
The benefits of using open-source tools include:
- Cost-Effectiveness: Open-source tools are usually free to use, meaning that organizations can rely on them without large upfront investments.
- Flexibility and Customization: Users can adapt open-source software to meet specific requirements thanks to customizable code.
- Community Support: A global user community often contributes to forums, documentation, and tutorials, aiding users in resolving issues or learning new techniques.
- Innovation: Many cutting-edge developments occur within the open-source community, leading to rapid advancements in analytics methodologies.
However, organizations choosing open-source options must often rely more on internal expertise for implementation and support. This necessitates a certain level of pre-existing knowledge within the organization to effectively leverage these tools.
"Choosing the right data analytics program can determine the success of data-driven initiatives within an organization. Companies must weigh the pros and cons of each solution to find the best fit for their unique situation."
In summary, both commercial software solutions and open-source tools play crucial roles in the ecosystem of data analytics. Each type brings distinct advantages and challenges, making it essential for organizations to analyze their specific needs in order to make informed software decisions.
Essential Algorithms in Data Analytics
Understanding essential algorithms in data analytics is a fundamental element of this article. Algorithms serve as the backbone of data analytics programs, determining how data is processed, analyzed, and interpreted. They allow professionals to derive meaningful insights from raw data, enhancing decision-making and operational strategies. In an era dominated by data, the capacity to effectively utilize these algorithms is imperative for organizations aiming to harness their data for competitive advantage.
Algorithms facilitate various analytical tasks by utilizing different learning techniques. They help in structuring data, predicting outcomes, and uncovering patterns. The choice of algorithm can significantly influence outcomes, which is why comprehending their core principles is crucial. Below is a detailed look at various types of algorithms, their functions, and applications.
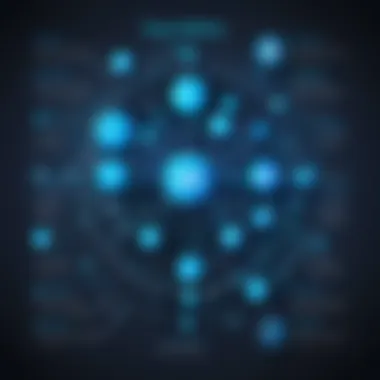
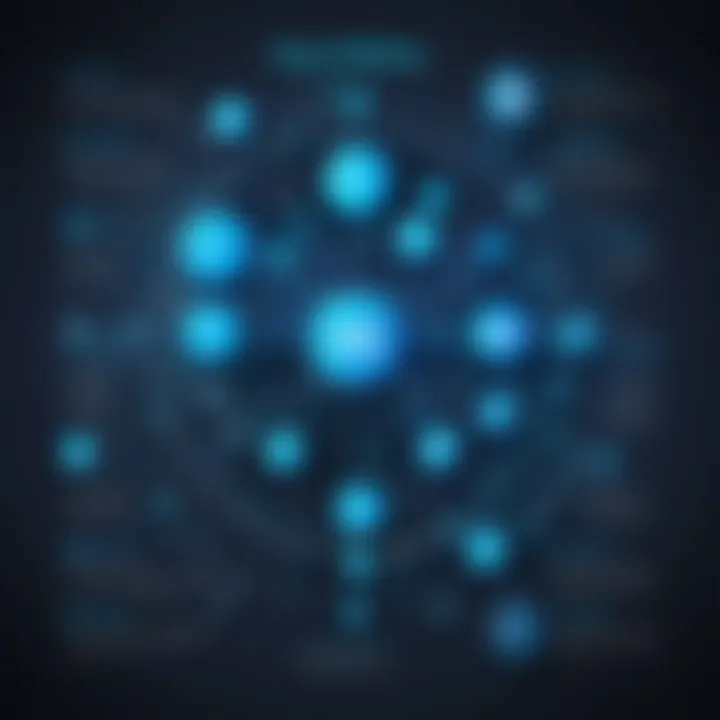
Supervised Learning Algorithms
Supervised learning algorithms are designed to identify patterns in data by using a labeled dataset. This type of learning requires input-output pairs where the input is known, and the desired output is already available. Common algorithms include linear regression, logistic regression, support vector machines, and decision trees.
Advantages of Supervised Learning:
- Provides accurate predictions based on historical data.
- Continuously improves as more labeled data is available.
- Generally easier to understand due to clear definitions of inputs and outputs.
When using supervised learning, practitioners can train models to predict outcomes in various scenarios, making it valuable in fields such as finance for credit scoring or in healthcare for treatment recommendations.
Unsupervised Learning Algorithms
Unsupervised learning, in contrast, deals with datasets that do not have labeled responses. The primary goal is to uncover hidden patterns without predefined outputs. Algorithms such as k-means clustering, hierarchical clustering, and principal component analysis are commonly used in this context.
Benefits of Unsupervised Learning:
- Helps in discovering groups or segments within data.
- Useful for data preprocessing and dimensionality reduction.
- Can enhance understanding of data structure, providing insights that may not be immediately apparent.
This approach is especially beneficial in areas like market research, where businesses seek to categorize customers or identify purchasing behaviors without predetermined categories.
Reinforcement Learning Approaches
Reinforcement learning algorithms function differently from both supervised and unsupervised categories. They involve agents that learn to make decisions by interacting with their environment. The algorithms use reward signals to evaluate the effectiveness of their actions. Popular methods include Q-learning and deep reinforcement learning.
Key Features of Reinforcement Learning:
- Focuses on learning through trial and error.
- Applicable in dynamic and complex environments, like game playing or robotics.
- Offers real-time feedback, allowing algorithms to adapt their strategies effectively.
In summary, the study of essential algorithms enriches the data analytics landscape, empowering professionals to leverage their data efficiently. Understanding these algorithms is a pivotal step toward deriving actionable insights and achieving strategic goals in any data-centric initiative.
Applications of Data Analytics Programs
In the realm of data analytics, applications play a pivotal role. They transform raw data into actionable insights, enabling businesses to measure progress, identify trends, and improve outcomes. Analysing how these applications function and their potential benefits forms a crucial part of understanding data analytics computer programs.
Introducing new applications in analytics often results in better strategic decisions that involve market positioning, operational efficiency, and customer engagement. As organizations strive to adapt in an ever-evolving data landscape, leveraging these applications becomes essential for maintaining a competitive edge.
Business Intelligence
Business intelligence (BI) refers to technologies and strategies used by companies to analyze data and present actionable information. BI applications harness data from various sources, enabling business leaders to make informed choices. For instance, tools like Tableau and Microsoft Power BI allow users to create visual reports. With a clear overview of performance metrics, organizations can swiftly pinpoint areas for improvement.
Moreover, BI tools facilitate real-time data analysis. This feature is significant in dynamic sectors like retail or finance, where rapid decision-making is vital. BI aids in spotting market shifts and responding accordingly. Using dashboards, stakeholders can monitor operations daily and adjust strategies based on current insights.
Predictive Analytics
Predictive analytics involves using statistical algorithms and machine learning techniques to identify the likelihood of future outcomes based on historical data. This application allows organizations to forecast trends, anticipate customer behavior, and allocate resources efficiently. For example, predictive models can analyze past purchasing data to predict future buying patterns.
Using platforms such as SAS or IBM Watson, organizations can mine vast datasets to glean patterns. These insights can lead to more precision in marketing efforts and product development, ultimately enhancing the customer's experience.
"Predictive analytics empowers businesses to transform data into foresight, better aligning their strategies with market demands."
Customer Insights and Segmentation
Understanding customer behavior is critical for any business. Customer insights enable companies to tailor their offerings and engagement efforts. By analyzing consumer data, organizations can identify segments and create personalized marketing strategies. This segmented approach often leads to higher conversion rates.
Programs like Google Analytics or Adobe Analytics facilitate this process. They provide capabilities for tracking customer interactions across various platforms, helping identify preferences and behaviors.
Key benefits of utilizing customer insights include:
- Improved customer retention
- Targeted advertising strategies
- Enhanced product development based on actual demand
By effectively leveraging these insights, businesses can not only enhance customer satisfaction but also foster long-term loyalty.
Challenges in Implementing Data Analytics Programs
Implementing data analytics programs is often more complex than it appears. The challenges associated with these implementations are significant and can hinder a company's ability to derive meaningful insights from their data. Addressing these challenges is crucial for successful integration of data analytics within an organization. Below, we will explore three primary obstacles: data quality issues, integration with existing systems, and the skill gap among employees.
Data Quality Issues
Data quality is one of the most pressing challenges in data analytics. Poor quality data can lead to inaccurate analyses, which in turn affects decision-making and strategic planning. Companies must ensure that the data they use is accurate, complete, and timely. This involves regular data validation and cleansing processes.
Common issues include:
- Inconsistent data formats
- Missing values
- Duplicate records
To mitigate these problems, businesses should invest in robust data governance practices. Clear protocols for data entry and maintenance can help maintain data integrity. Furthermore, employing tools specifically designed for data quality management can greatly enhance the accuracy of analytics results.

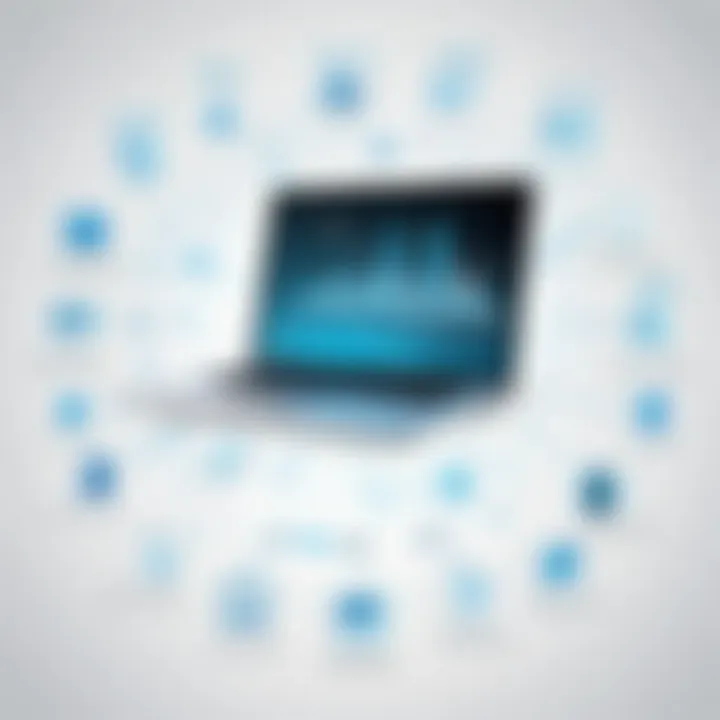
Integration with Existing Systems
Integrating data analytics programs with existing systems can pose considerable difficulties. Organizations typically rely on numerous software solutions and databases that may not be easily compatible with new analytics tools. This fragmentation can lead to silos of data, making it challenging to gain a unified view of information across the organization.
Successful integration requires:
- Comprehensive understanding of current systems and architecture
- Strategic planning for implementation
- Ongoing collaboration between IT and data analytics teams
Failure to address integration issues can result in wasted resources and lost opportunities. Thus, it is essential to prioritize seamless connectivity between systems to ensure effective data flow and analysis.
Skill Gap and Training Requirements
A significant barrier to the successful implementation of data analytics is the skill gap within the workforce. Many organizations find that their employees lack the necessary knowledge to effectively utilize advanced analytics tools. This can lead to underutilization of the technology and prevent organizations from unlocking its full potential.
Addressing this gap involves:
- Investing in training programs: Organizations should offer resources for employees to gain skills in data analysis and the specific tools being used.
- Encouraging a culture of learning: Fostering an environment where continuous learning is valued will help employees adapt to new technologies more readily.
- Hiring specialized talent: In some cases, it may be necessary to bring in external professionals who possess the required skills.
Future Trends in Data Analytics Software
Data analytics is evolving rapidly, with emerging technologies continuously reshaping the landscape. Understanding future trends in data analytics software is crucial for organizations that want to remain competitive in a data-driven world. The integration of new technologies such as artificial intelligence and real-time capabilities is expected to redefine how businesses approach data analysis. These advancements not only enhance the precision of insights but also streamline workflows and improve overall efficiency. Staying informed about these trends can help businesses make strategic decisions and align their operations with best practices in data analytics.
Artificial Intelligence Integration
The integration of artificial intelligence (AI) into data analytics software is perhaps the most significant trend shaping the industry. AI technologies empower analytics software to perform complex data analysis at unprecedented speeds. This allows for more sophisticated insights that are not only automated but also predictive in nature.
The benefits of AI in data analytics include:
- Enhanced Data Processing: AI algorithms can analyze massive datasets quickly, identifying patterns and trends that may not be visible to human analysts.
- Predictive Analytics: AI adds the ability to forecast future trends based on historical data, giving businesses a significant advantage in planning.
- Natural Language Processing: AI tools can interpret human language, allowing for more intuitive interaction with data analytics software.
Nonetheless, the integration of AI also raises considerations about data accuracy and the ethical implications of automated decision-making. Companies must ensure that AI systems operate transparently and fairly to avoid bias in the data analysis process.
Real-Time Analytics Capabilities
Another critical trend is the growing emphasis on real-time analytics. As businesses strive to make decisions faster, the ability to access and analyze data in real-time becomes a vital feature. This capability allows organizations to react quickly to changing market conditions, customer behaviors, and operational inefficiencies.
Key benefits of real-time analytics include:
- Improved Decision-Making: Instant insights enable organizations to make informed choices without delay.
- Agility in Operations: Real-time data helps companies adapt their strategies quickly, maintaining relevance in a fast-paced environment.
- Enhanced Customer Experience: Immediate awareness of customer interactions enables businesses to tailor their services effectively.
To implement real-time analytics successfully, companies need to invest in the appropriate technology infrastructure and ensure that their data sources are reliable and current. With the right setup, organizations can leverage real-time analytics to gain a competitive edge, driving innovation and business growth.
"The future of data analytics lies in leveraging AI and real-time capabilities to uncover insights that were previously unattainable."
Evaluating Data Analytics Software
Evaluating data analytics software is a crucial step for organizations aiming to leverage data in their decision-making processes. The selection of the right tool can significantly influence the overall effectiveness of data analytics strategies. Each program comes with unique functionalities, strengths, and weaknesses. Therefore, understanding how to evaluate these tools ensures that businesses invest in solutions that meet their specific needs.
As data analytics continues to evolve, it becomes clearer that companies cannot afford to make hasty choices in software. An inappropriate decision may result in wasted resources, including time and money, as well as missed opportunities for insights that could drive growth. By carefully assessing options, organizations can align their analytics tools with both current goals and future ambitions.
Criteria for Selection
When selecting data analytics software, several key criteria may guide the decision-making process. Understanding these can facilitate a more informed choice:
- Functionality: The software must meet the specific demands of the business. This includes support for data integration, data cleaning, and specific analytical capabilities.
- Usability: User experience plays a role in adoption. Intuitive interfaces can reduce training time and improve productivity.
- Scalability: A tool should be capable of growing with the organization. Capacity to handle increasing data volumes is essential for future-proofing the investment.
- Cost: Evaluating the pricing structure is fundamental. Some programs may have hidden costs that could affect the budget in the long run.
- Support and Community: Good customer support and an active user community can be vital for troubleshooting and learning.
Cost-Benefit Analysis
In any decision regarding software selection, conducting a cost-benefit analysis is essential. This process helps in gauging the value the software can bring against the total costs involved.
- Direct Costs: Analyze the purchase price, licensing fees, and maintenance costs.
- Indirect Costs: Consider training expenses, potential downtime during transition, and the cost related to adjustments in workflows.
- Benefits: Identify the potential improvements in efficiency, increased accuracy of insights, and the ability to make better-informed decisions.
Establishing quantifiable metrics for these benefits can also aid in justifying the investment.
"Effective evaluation and analysis can transform the perception of data analytics from just a support function into a strategic partner in driving business success."
The process of evaluating data analytics software requires careful consideration of multiple factors. By prioritizing needs and analyzing costs against expected benefits, organizations are more likely to choose the right tools that allow them to harness the power of data effectively.
Epilogue
In the realm of data analytics, the conclusion serves as a crucial point of synthesis and reflection. It encapsulates the key insights presented throughout the article, emphasizing the profound importance of data analytics in modern enterprises. As businesses continue to navigate a landscape defined by rapid technological evolution, understanding data analytics becomes essential.
The Importance of Data Analytics in Modern Enterprises
Data analytics provides a strategic advantage that is necessary for sustaining competitive edge. By leveraging data analytics, companies can transform raw data into actionable insights. This transformation influences multiple aspects, from operational efficiency to enhanced customer experiences.
The following elements highlight the significance of data analytics in todayβs corporate environment:
- Informed Decision-Making: Data analytics equips decision-makers with empirical evidence, allowing for strategic choices based on factual data rather than intuition.
- Increased Efficiency: Through data analytics, organizations can identify inefficiencies within processes and workflows, leading to cost reduction and improved productivity.
- Enhanced Customer Understanding: Companies can analyze customer behavior and preferences, which helps in tailoring products and services to meet specific needs, ultimately driving customer satisfaction and loyalty.
- Predictive Capabilities: Advanced analytics tools enable predictive modeling, aiding organizations in foreseeing trends and preparing for future demands.
"In the age of information, data analytics is no longer a luxury but a necessity for survival and growth."
Moreover, organizations face challenges in adopting data analytics, including data quality issues and the integration of systems. Addressing these hurdles is a critical consideration for leveraging the full benefits of data analytics.
The future of data analytics promises deeper integration with artificial intelligence and improved real-time analytics capabilities. These trends indicate that businesses will increasingly rely on data-driven strategies to thrive.
In summary, the conclusion of this exploration affirms that data analytics is instrumental in crafting innovative and effective strategies. By incorporating analytics into their core operations, enterprises can adapt and grow in an increasingly competitive market.